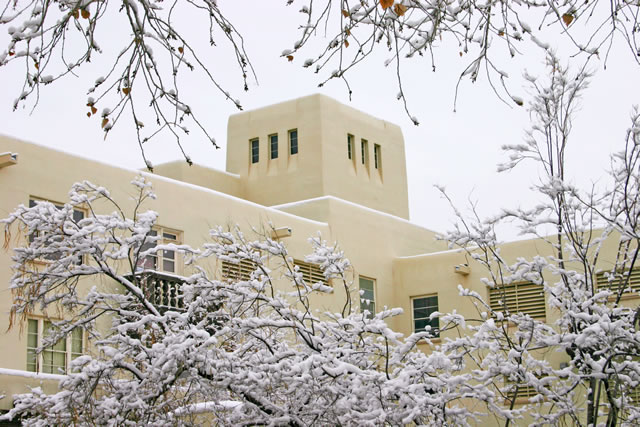
Organization, Information and Learning Sciences ETDs
Publication Date
Summer 8-1-2023
Abstract
Chapter 2 introduces an innovative method integrating Geographic Information Systems and Machine Learning to evaluate educational policy outcomes. This approach overcomes the limitations of traditional statistical methods and effectively analyzes complex relationships between educational data and demographic factors.
The study in Chapter 3 developed a prediction model for school report cards in New Mexico using census data and applying the methods described in Chapter 2. The accurate predictions suggested that the school accountability system disproportionately assigned failing grades to schools serving minoritized students. The study also confirmed that status measures depend more on demographics than growth measures.
The study in Chapter 4 used eye-tracking data to analyze collaborative decision-making during complex problem-solving. It revealed relationships between students’ status and excluding agency and predicted, through cross-recurrence analysis and machine learning, instances of excluding agency with 77% accuracy. The study further reveals the evolution of shared and excluding agency dynamics over time.
Degree Name
Organization, Information and Learning Sciences
Level of Degree
Doctoral
Department Name
Organization, Information & Learning Sciences
First Committee Member (Chair)
Vanessa Svihla
Second Committee Member
Karl Benedict
Third Committee Member
Scott Hughes
Fourth Committee Member
Meeko Oishi
Language
English
Keywords
School Accountability, Geographic Information Systems, Machine Learning, Random Forest, Quantitative Critical Theory, Collaboration in Learning Groups, Excluding Agency, Cross-Recurrence Quantification Analysis, Eye-Trackers
Document Type
Dissertation
Recommended Citation
Jiménez, Manuel J.; Vanessa Svihla; Karl Benedict; Victor Law; and Meeko Oishi. "Advanced Quantitative Approaches to Unveil Social Inequities in Educational Contexts." (2023). https://digitalrepository.unm.edu/oils_etds/68
Included in
Disability and Equity in Education Commons, Education Policy Commons, Other Social and Behavioral Sciences Commons, Social Justice Commons, Social Statistics Commons