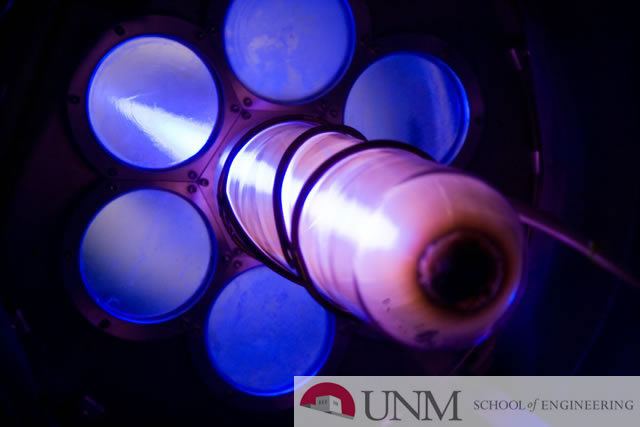
Electrical and Computer Engineering ETDs
Publication Date
Fall 10-28-2024
Abstract
As the need for “data on demand” increases, users are moving to hybrid communication systems to increase data throughput. Users in both commercial and government sectors are looking to integrate laser communication (lasercom) and W/V band (81-86 GHz / 71-76 GHz) solutions onto their platforms. These systems are subject to attenuation due to weather and atmospheric conditions. Employing a hybrid communication system requires knowledge of which system to utilize more heavily in given atmospheric conditions. This research investigates means to develop channel prediction algorithms for hybrid systems using weather imagery. Convolutional Neural Networks (CNNs) were adapted and trained with these weather products and then utilized to develop a decision algorithm that can predict which system will provide the highest performance in a given atmospheric condition. The decision algorithms were developed and tested on a terrestrial link provided by the Air Force Research Laboratory, Space Vehicles Directorate (AFRL/RV).
Keywords
Laser communications, convolutional neural nets, hybrid communication systems
Document Type
Dissertation
Language
English
Level of Degree
Doctoral
Department Name
Electrical and Computer Engineering
First Committee Member (Chair)
Christos Christodoulou
Second Committee Member
Jane Lehr
Third Committee Member
Trilce Estrada
Fourth Committee Member
Richard Scott Erwin
Fifth Committee Member
Thomas Farrell
Recommended Citation
Smith, Julie C.. "CHANNEL SELECTION OF A HYBRID COMMUNICATION SYSTEM USING MACHINE LEARNING." (2024). https://digitalrepository.unm.edu/ece_etds/694