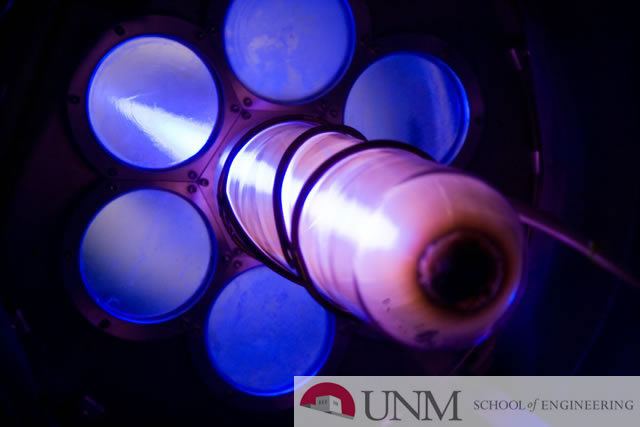
Electrical and Computer Engineering ETDs
Publication Date
Fall 12-15-2024
Abstract
Modelling atmospheric loss is fundamental to implementation of future mm-wave terrestrial and earth space communication links. Atmospheric losses have traditionally been estimated by calculating the contributions of individual weather phenomena through in-situ meteorological measurements and statistically derived models for estimating localized atmospheric water content. Collecting data for all points along the propagation path and calculating the physical effects of gases, clouds, and precipitation individually is both time consuming and impractical for large-scale use cases.
In response to these present limitations, this work proposes the use of novel deep learning models for estimating atmospheric losses from tabular meteorological sensor data and doppler weather radar images. These models were validated on a multi-year propagation measurement campaign operating at 72 GHz and when combined with a natural gradient boosting algorithm to provide a unique probabilistic estimator. This new fusion deep learning and gradient boosting model can predict atmospheric loss based on current meteorological conditions along with the model’s confidence.
Keywords
Propagation, V-band, Deep Learning
Document Type
Dissertation
Language
English
Degree Name
Electrical Engineering
Level of Degree
Doctoral
Department Name
Electrical and Computer Engineering
First Committee Member (Chair)
Christos Christodoulou
Second Committee Member
Mark Gilmore
Third Committee Member
Trilce Estrada
Fourth Committee Member
Steven Lane
Recommended Citation
Gesner, Ralph Lyndon. "Modelling V-band Atmospheric Loss with Deep Learning." (2024). https://digitalrepository.unm.edu/ece_etds/686