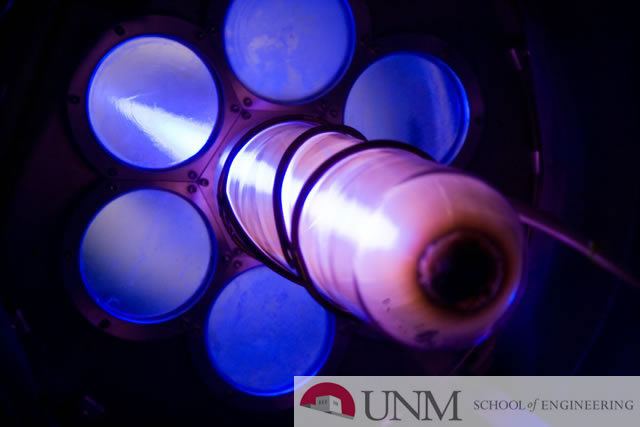
Electrical and Computer Engineering ETDs
Publication Date
Fall 9-20-2024
Abstract
Electric load prediction (ELP) can support smart grid (SG) goals such as reliability and efficiency. In ELP, we predict the electricity demand at aggregated levels which is vital for the proper functioning of SG and keeping a balance between load and supply demand. ELP can be categorized as very short-term, short-term, medium-term, and long-term ELP. In this dissertation, we propose multi-output Gaussian processes (MOGP) for hourly day-ahead short-term ELP. We investigate different feature scaling techniques and prediction accuracy metrics with comprehensive details. Mean absolute percentage error (MAPE) is used as a prediction accuracy metric. We proposed four methods: MOGP, MOGP with multiple kernel learning (MKL), multi-output sparse Gaussian processes (MOSGP), and MOSGP with MKL. We compared the performance of the proposed methods with the persistence method, multiple linear regression (MLR), and support vector regression (SVR). The results show that the four proposed methods outperform the persistence method, MLR, and SVR.
Keywords
Smart grid
Document Type
Dissertation
Language
English
Degree Name
Electrical Engineering
Level of Degree
Doctoral
Department Name
Electrical and Computer Engineering
First Committee Member (Chair)
Prof. Balasubramaniam Santhanam
Second Committee Member
Prof. Mark Gilmore
Third Committee Member
Prof. Li Luo
Fourth Committee Member
Prof. Charles Fleddermann
Fifth Committee Member
Prof. Ashutosh Dutta
Recommended Citation
Ghasempour, Alireza. "Integrating Machine Learning, Data Fusion, and Statistical Methods to Predict Electric Load in Smart Grids." (2024). https://digitalrepository.unm.edu/ece_etds/687