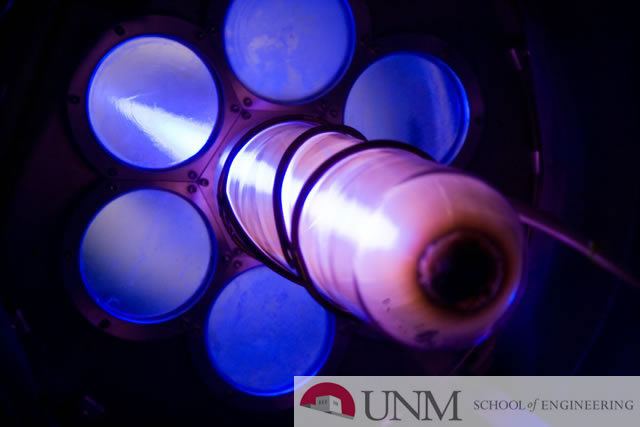
Electrical and Computer Engineering ETDs
Publication Date
Summer 7-10-2024
Abstract
This thesis presents a system for analyzing student activities during class sessions to gain insights into the learning process. A dataset consisting of 14 screen recordings, with 2 videos labeled across two stages, was used for training, validation, and testing. The methodology employs adaptive sampling, initially at 10 frames per minute to identify active regions, followed by detailed analysis at 1 frame per second using OCR to detect typing activities through character changes. The results demonstrate over a 50% reduction in computational load while maintaining high accuracy in detecting student engagement. A total of 26 hours of screen capture videos were processed, with AWS Textract achieving 87% accuracy, Tesseract 80%, EasyOCR 76%, and MMOCR 72%. By analyzing activity and typing patterns, the system significantly reduces the need for manual video review, providing valuable insights into how students interact with computers, the time spent by each group, and periods of engagement, thereby enhancing our understanding of student interaction dynamics.
Keywords
Video Analysis, Screen Recordings, Adaptive Sampling, Student Engagement, Multilayered Dataset, Typing Detection
Document Type
Thesis
Language
English
Degree Name
Computer Engineering
Level of Degree
Masters
Department Name
Electrical and Computer Engineering
First Committee Member (Chair)
Marios Pattichis
Second Committee Member
Ramiro Jordan
Third Committee Member
Venkatesh Jatla
Recommended Citation
Egala, Ugesh. "Adaptive Screen Capture Video Analysis." (2024). https://digitalrepository.unm.edu/ece_etds/663
Included in
Educational Assessment, Evaluation, and Research Commons, Electrical and Computer Engineering Commons