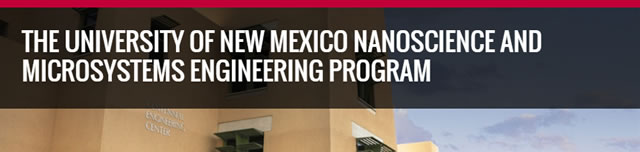
Nanoscience and Microsystems ETDs
Publication Date
Summer 7-31-2022
Abstract
Nanotechnology promises to revolutionize many areas of applied science including materials, synthetic biology, and medicine. Devices may consist of solution-phase information processing systems or molecular robots. Of particular interest are DNA-based systems due to their composability and relatively simple interactions that are suited to bottom-up design. This work introduces high-level designs of robust control mechanisms for nanoscale devices with immediate applications in molecular computing, synthetic biology, and DNA robotics.
Nanoscale systems are dominated by probabilistic chemical behavior, so engineers must take careful consideration to produce predictable systems. Probabilistic behavior in chemical reaction networks (CRNs) yields deterministic evolution of species concentrations in systems with sufficiently large numbers of chemicals, which can be used to emulate precise mathematical functions of variables whose values are encoded as concentrations. In part, this work presents a CRN that implements a simple neural network that autonomously approximates supervised backpropagation learning, which can be used for innumerable chemical learning applications. In contrast, systems with low molecular counts experience exaggerated effects of stochasticity, which is common in biological and synthetic chemical systems. For example, as genes are expressed in individual cells, there may be on the order of hundreds or thousands of a given species; a molecular walking robot may have as few as one leg and two possible locations for its next step. This produces nondeterministic dynamics and unique design problems. To address nanoscale robotics, we explore the effect of geometrical constraints on teams of coupled surface-bound random walkers to increase their propensity for directed motion. Finally, this work presents CRNs capable of population-level consensus in stochastic systems, producing finite system states with tunable control over state choices and transitions.
Keywords
chemical reaction network, molecular computing, nanotechnology, DNA
Document Type
Dissertation
Language
English
Degree Name
Nanoscience and Microsystems
Level of Degree
Doctoral
Department Name
Nanoscience and Microsystems
First Committee Member (Chair)
Matthew R. Lakin
Second Committee Member
Darko Stefanovic
Third Committee Member
Lydia Tapia
Fourth Committee Member
William Bricker
Recommended Citation
Arredondo, David. "Control Mechanisms for Adaptive Nanoscale Devices." (2022). https://digitalrepository.unm.edu/nsms_etds/67