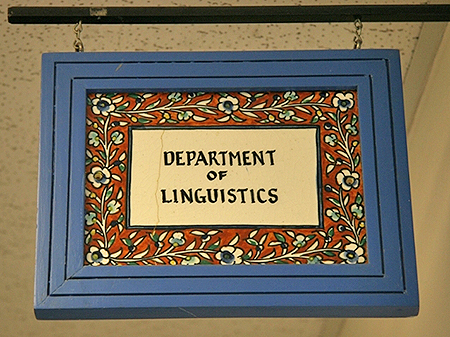
Linguistics ETDs
Publication Date
5-1-2010
Abstract
Transforming an acoustic signal to words is the gold standard in automatic speech recognition. While recognizing that orthographic transcription is a valuable technique for comparing speech recognition systems without respect to application, it must also be recognized that transcription is not something that human beings do with their language partners. In fact, transforming speech into words is not necessary to emulate human performance in many contexts. By relaxing the constraint that the output of speech recognition be words, we might at the same time effectively relax the bias toward writing in speech recognition research. This puts our work in the camp of those who have argued over the years that speech and writing differ in significant ways. This study explores two hypotheses. The first is that a large vocabulary continuous speech recognition (LVCSR) system will perform more accurately if it were trained on syllables instead of words. Though several researchers have examined the use of syllables in the acoustic model of an LVCSR system, very little attention has been paid to their use in the language model. The second hypothesis has to do with adding a post-processing component to a recognizer equipped with a syllable language model. The first step is to group words that seem to mean the same thing into equivalence classes called concepts. The second step is to insert the equivalence classes into the output of a recognizer. The hypothesis is that by using this concept post-processor, we will achieve better results than with the syllable language model alone. The study reports that the perplexity of a trigram syllable language model drops by half when compared to a trigram word language model using the same training transcript. The drop in perplexity carries over to error rate. The error rate of a recognizer equipped with syllable language model drops by over 14% when compared with one using a word language model. Nevertheless, the study reports a slight increase in error rate when a concept post-processor is added to a recognizer equipped with a syllable language model. We conjecture that this is the result of deterministic mapping from syllable strings to concepts. Consequently, we outline a probabilistic mapping scheme from concepts to syllable strings.
Language
English
Keywords
computational linguistics, computer science, speech recognition, language model, syllables, concepts, linguistics
Document Type
Dissertation
Degree Name
Computational Linguistics
Level of Degree
Doctoral
Department Name
Department of Linguistics
First Committee Member (Chair)
Croft, William
Second Committee Member
Smith, Caroline
Third Committee Member
Wooters, Charles
Recommended Citation
De Palma, Paul A.. "Syllables and Concepts in Large Vocabulary Speech Recognition." (2010). https://digitalrepository.unm.edu/ling_etds/9
Comments
Submitted by Paul De Palma (depalma@gonzaga.edu) on 2010-04-16T02:14:09Z No. of bitstreams: 1 Paul_De_Palma_4-15-10_Final.pdf: 3175052 bytes, checksum: 557ea5a18cbf44c469919553d0ecfeea (MD5), Approved for entry into archive by Doug Weintraub(dwein@unm.edu) on 2010-06-25T22:12:59Z (GMT) No. of bitstreams: 1 Paul_De_Palma_4-15-10_Final.pdf: 3175052 bytes, checksum: 557ea5a18cbf44c469919553d0ecfeea (MD5), Made available in DSpace on 2010-06-25T22:13:03Z (GMT). No. of bitstreams: 1 Paul_De_Palma_4-15-10_Final.pdf: 3175052 bytes, checksum: 557ea5a18cbf44c469919553d0ecfeea (MD5)