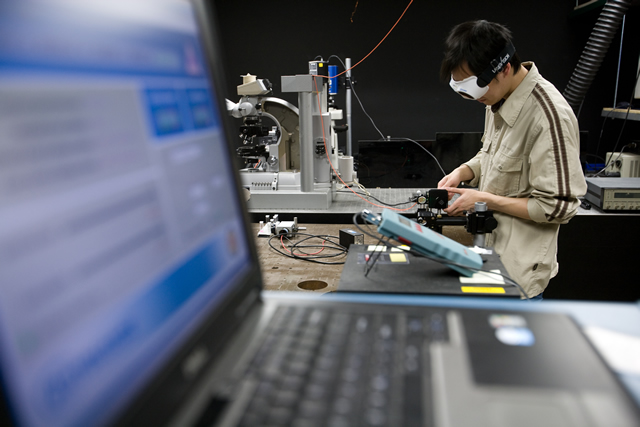
Electrical & Computer Engineering Faculty Publications
Document Type
Article
Publication Date
6-22-2003
Abstract
Machine learning research has largely been devoted to binary and multiclass problems relating to data mining, text categorization, and pattern/facial recognition. Recently, popular machine learning algorithms, including support vector machines (SVM), have successfully been applied to wireless communication problems. The paper presents a multiclass least squares SVM (LS-SVM) architecture for direction of arrival (DOA) estimation as applied to a CDMA cellular system. Simulation results show a high degree of accuracy, as related to the DOA classes, and prove that the LS-SVM DDAG (decision directed acyclic graph) system has a wide range of performance capabilities. The multilabel capability for multiple DOAs is discussed. Multilabel classification is possible with the LS-SVM DDAG algorithm presented.
Publisher
IEEE
Publication Title
IEEE Antennas and Propagation Society International Symposium
ISSN
0-7803-7846-6
First Page
57
Last Page
60
DOI
10.1109/APS.2003.1217400
Language (ISO)
English
Sponsorship
IEEE
Keywords
Data mining, Direction of arrival estimation, Least squares approximation
Recommended Citation
Abdallah, Chaouki T.; Judd A. Rohwer; and Christos G. Christodoulou. "Least squares support vector machines for direction of arrival estimation." IEEE Antennas and Propagation Society International Symposium (2003): 57-60. doi:10.1109/APS.2003.1217400.