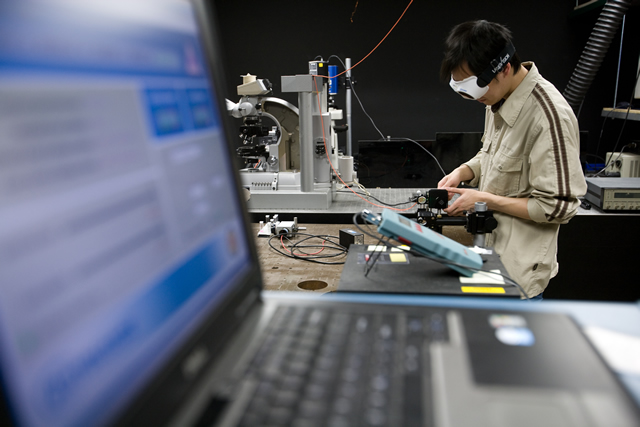
Electrical & Computer Engineering Faculty Publications
Document Type
Article
Publication Date
12-11-1996
Abstract
A nonlinear observer for a general class of single-output nonlinear systems is proposed based on a generalized dynamic recurrent neural network (DRNN). The neural network (NN) weights in the observer are tuned online, with no off-line learning phase required. The observer stability and boundness of the state estimates and NN weights are proven. No exact knowledge of the nonlinear function in the observed system is required. Furthermore, no linearity with respect to the unknown system parameters is assumed. The proposed DRNN observer can be considered as a universal and reusable nonlinear observer because the same observer can be applied to any system in the class of nonlinear systems.
Publisher
IEEE
Publication Title
Proceedings of the 35th IEEE Decision and Control
ISSN
0-7803-3590-2
Issue
1
First Page
949
Last Page
954
DOI
10.1109/CDC.1996.574590
Language (ISO)
English
Sponsorship
IEEE
Keywords
Chaos, Erbium, Nonlinear systems
Recommended Citation
Abdallah, Chaouki T.; Young H. Kim; and Frank L. Lewis. "Nonlinear observer design using dynamic recurrent neural networks." Proceedings of the 35th IEEE Decision and Control (1996): 949-954. doi:10.1109/CDC.1996.574590.