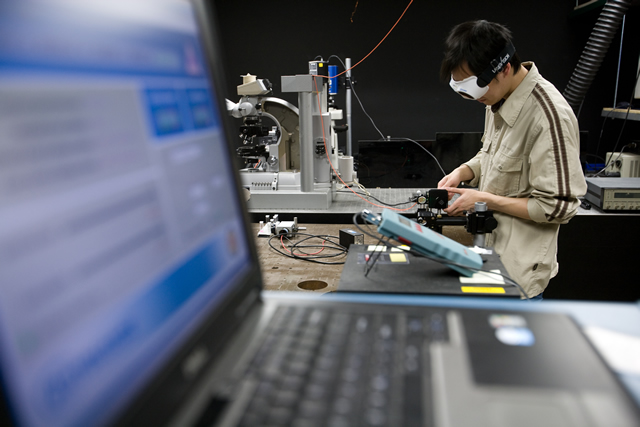
Electrical & Computer Engineering Faculty Publications
Document Type
Article
Publication Date
4-26-2012
Abstract
This paper shows how probabilistic methods and statistical learning theory can provide approximate solutions to “difficult” control problems. The paper also introduces bootstrap learning methods to drastically reduce the bound on the number of samples required to achieve a performance level. These results are then applied to obtain more efficient algorithms which probabilistically guarantee stability and robustness levels when designing controllers for uncertain systems. The paper includes examples of the applications of these methods.
Language (ISO)
English
Keywords
Statistical Learning, Radamacher bootstrap, Robust Control, Sample Complexity, NP-hard problems, Decidability theory
Recommended Citation
Abdallah, Chaouki T.; M. Ariola; and V. Koltchinskii. "Application of Statistical Learning Control to the Design of a Fixed-Order Controller for a Flexible Beam." (2012). https://digitalrepository.unm.edu/ece_fsp/141