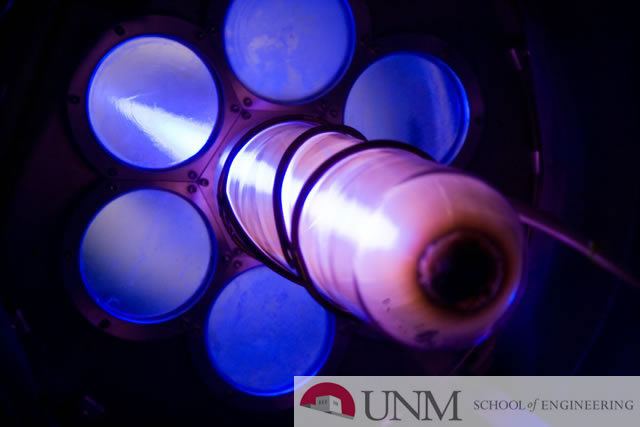
Electrical and Computer Engineering ETDs
Publication Date
Fall 12-15-2024
Abstract
Non-Gaussian uncertainty frequently arises in learning and control problems involving stochastic dynamical systems, particularly in autonomous vehicles, UAVs, satellites, and robotics. In this dissertation, we propose a new framework that leverages characteristic functions that provides a frequency-domain representation of random variables. The dissertation is structured into three key areas. First, we address model-based stochastic optimal control for linear systems with non-Gaussian noise, demonstrating that characteristic functions can be used to enforce chance constraints and control systems toward desired distributions. Second, we explore data-driven stochastic control, utilizing empirical characteristic functions to handle systems with unknown disturbances. In addition, we derive several metrics of the cost distribution through characteristic functions, which facilitates further exploration in reinforcement learning. Finally, we utilize characteristic functions in neural network verification by propagating by propagating distributions through ReLU activation functions. While this analytical propagation shows promise, we reveal its limitations in higher dimensions and propose a sampling-based approach to verification that maintains guarantees. The core novelty of this dissertation is the creation of a set of mathematical tools and methods that can be used to address difficult problems in stochastic optimal control, neural net verification, and reinforcement learning. These methods and tools are designed to facilitate learning, propagation, and exploitation of uncertainty in autonomous dynamical system, well beyond state-of-the-art approaches.
Keywords
Stochastic Optimal Control, Uncertainty Propagation, Non-Gaussian Disturbances, Characteristic Functions, Neural Network Verification, Reinforcement Learning
Sponsors
National Science Foundation, NASA, Air Force
Document Type
Dissertation
Language
English
Degree Name
Electrical Engineering
Level of Degree
Doctoral
Department Name
Electrical and Computer Engineering
First Committee Member (Chair)
Meeko M.K. Oishi
Second Committee Member
Rafael Fierro
Third Committee Member
Ali Bidram
Third Advisor
Claus Danielson
Fourth Committee Member
Panagiotis Tsiotras
Fifth Committee Member
Sean Phillips
Recommended Citation
Sivaramakrishnan, Vignesh. "Theory and algorithms to learn, propagate, and exploit uncertainty for stochastic optimal control of dynamical systems." (2024). https://digitalrepository.unm.edu/ece_etds/693
Included in
Controls and Control Theory Commons, Control Theory Commons, Dynamical Systems Commons, Dynamic Systems Commons, Navigation, Guidance, Control and Dynamics Commons, Probability Commons, Space Vehicles Commons, Systems Engineering and Multidisciplinary Design Optimization Commons