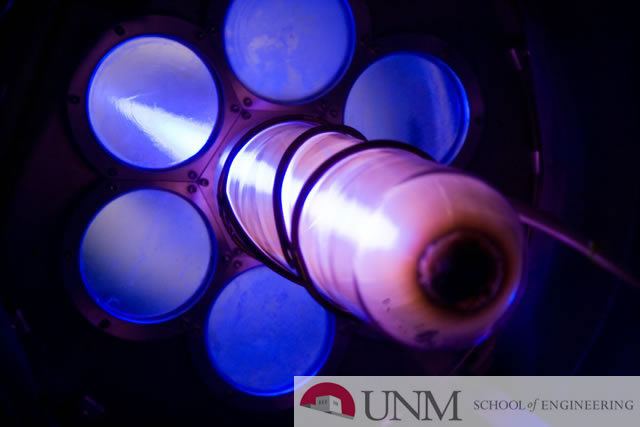
Electrical and Computer Engineering ETDs
Publication Date
Fall 11-14-2023
Abstract
The contemporary GPS infrastructure plays a vital role in advancing Position, Navigation, and Timing (PNT) applications across various sectors, including transportation, commerce, finance, and aviation. To provide a robust and authenticated infrastructure for users, it is important to develop a methodology for verifying the authenticity of satellite navigation signals, especially in civilian applications using the C/A code. This thesis explores Machine Learning (ML) techniques, focusing on the analysis of Global Navigation Satellite System (GNSS) protocol messages like UBX and NMEA to detect anomalies in receiver and satellite behavior, indicative of potential spoofing. The research demonstrates the deployment of Machine Learning models on a specialized Xilinx Kria KV260 FPGA hardware platform, enabling real-time solutions for signal authentication that can be readily deployed in the field, effectively addressing the need for signal security and validation.
Keywords
GPS Spoofing Detection, Machine Learning in PNT, GNSS Protocol Analysis, Signal Authentication, Machine Learning Models Deployment in Hardware Xilinx Kria KV260 FPGA, Real-Time Anomaly Detection
Document Type
Thesis
Language
English
Degree Name
Computer Engineering
Level of Degree
Masters
Department Name
Electrical and Computer Engineering
First Committee Member (Chair)
Dr. Eirini Eleni Tsiropoulou
Second Committee Member
Dr. James Aarestad
Third Committee Member
Mr. Brian Zufelt
Recommended Citation
Morales Chacon, Clarizza. "Design, Optimization, and Hardware Deployment of a Deep Learning Model for GPS Spoofing Detection using GNSS Satellite Receiver Protocol Data." (2023). https://digitalrepository.unm.edu/ece_etds/626