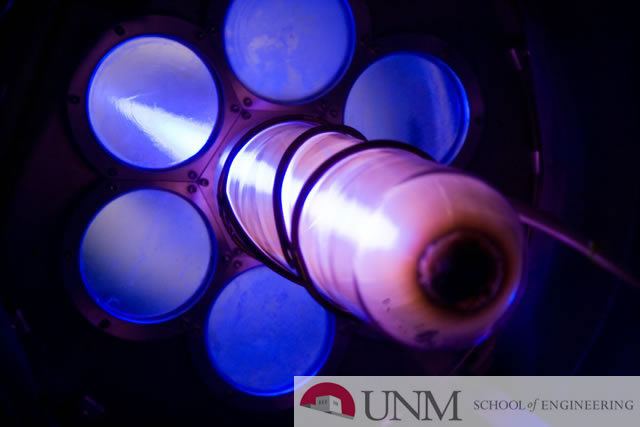
Electrical and Computer Engineering ETDs
Publication Date
Fall 10-8-2020
Abstract
In this thesis, we present a novel socio-physical human orchestration framework to deal with the increasing complexity of smart city environments, by capitalizing on recent advances in game theory and reinforcement learning, with the goal of solving the efficient management of a smart city while improving the quality of life for the humans living in said smart city. This problem has become more challenging as the smart cities have become more complicated and complex with new technologies. In the proposed framework, each human selects a Point of Interest (PoI) that it wants to visit. This selection is performed by acting as stochastic learning automaton, which evaluates the socio-physical conditions of the environment while learning from its previous experiences. As a result, those humans that have selected a specific PoI to visit, "compete" with each other in order to finally perform their visit. The humans' behavior is studied as a non-cooperative game among them, through adopting the theory of minority games. The resulting Nash equilibrium point classifies which of the humans that finally visit each PoI. A low complexity reinforcement learning based algorithm is used achieve the overall framework. Finally, a detailed set of numerical and comparative results are shown to provide information on the efficiency of the approach.
Document Type
Thesis
Language
English
Degree Name
Computer Engineering
Level of Degree
Masters
Department Name
Electrical and Computer Engineering
First Committee Member (Chair)
Eirini Eleni Tsiropoulou
Second Committee Member
Jim Plusquelic
Third Committee Member
Payman Zarkesh-Ha
Recommended Citation
Patrizi, Nathan. "Socio-physical Human Orchestration in Smart Cities." (2020). https://digitalrepository.unm.edu/ece_etds/495