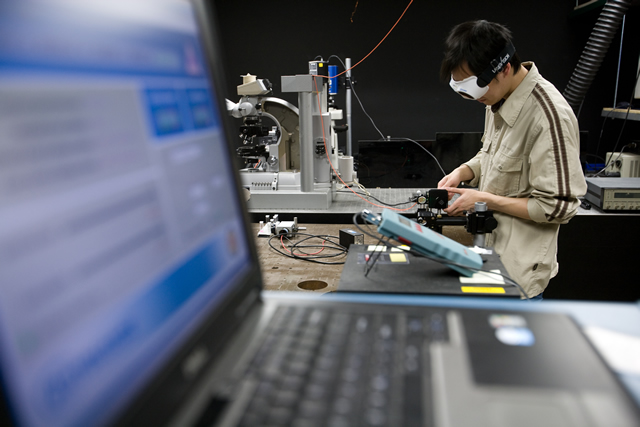
Computer Science ETDs
Publication Date
Winter 12-17-2021
Abstract
This dissertation addresses gaps in artificial intelligence-based computer vision tasks in the medical image processing field. We demonstrate effective methods for standardizing and augmenting digital fundus photographs so that robust convolutional neural network-based systems can perform high-throughput disease classification and generalize to never-before-seen data from novel camera technologies, scaling with the changing hardware landscape, as well as keeping up with vast amount of incoming data from the ever-increasing population. We also tackle the problem of discovering relevant samples in an unlabeled cohort of image data, thus widening the bottleneck to all downstream supervised machine learning tasks.
Language
English
Keywords
Artificial Intelligence, Biomedical Engineering, Data Discovery, Diabetic Retinopathy, Machine Learning
Document Type
Dissertation
Degree Name
Computer Science
Level of Degree
Doctoral
Department Name
Department of Computer Science
First Committee Member (Chair)
Trilce Estrada
Second Committee Member
Shuang Luan
Third Committee Member
Abdullah Mueen
Fourth Committee Member
Manel Martinez-Ramon
Fifth Committee Member
Peter Soliz
Recommended Citation
Benson, Jeremy Richard. "Systems and Methods for Scalable Retinal Screening Programs." (2021). https://digitalrepository.unm.edu/cs_etds/122