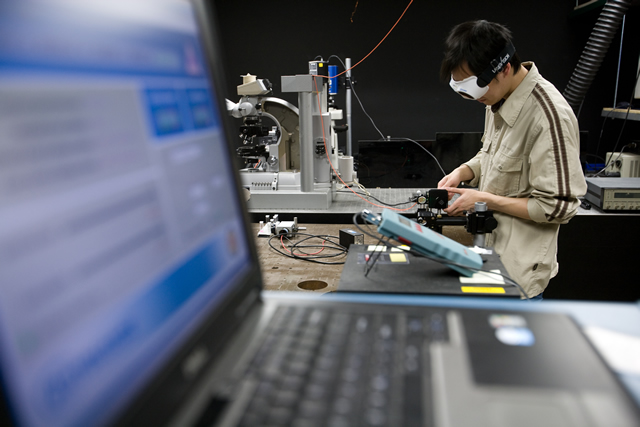
Computer Science ETDs
Publication Date
Summer 7-15-2022
Abstract
The current seismic data processing pipeline is surprisingly human-dependent. With the rapid increase of seismic-sensor data availability, all manual data processing approaches fail to detect, classify, and analyze seismic activity within a reasonable amount of time. An automated, fast, and reliable seismic data processing pipeline is desired for the meaningful analysis of massive seismic datasets. In this thesis, we show how advanced time-series data-mining and machine learning techniques can be leveraged to resolve this issue. We precisely focus on seismic activity detection, classification, and inspection using our techniques that would help us better understand the surrounding earth structure, earthquake evaluation, and seismic monitoring
In this dissertation, (a) we demonstrate a semi-supervised motif discovery algorithm that forms a nearest neighbor graph to discover novel seismic events from static continuous waveforms. (b) We exhibit a seismic data repository system that can extract thousands of seismic waveforms including annotations using complex queries within seconds. (c) We design and implement a hierarchical neural network that can predict seismic depth from seismograms and classify deep and shallow earthquakes with 86.5% F1 score.
Keywords
Machine learning, Data mining, Seismology, Explosion monitoring, Time series
Document Type
Dissertation
Degree Name
Computer Science
Level of Degree
Doctoral
Department Name
Department of Computer Science
First Committee Member (Chair)
Abdullah Mueen
Second Committee Member
Shuang Luan
Third Committee Member
Huiping Cao
Fourth Committee Member
Trilce Estrada
Fifth Committee Member
Jonathan MacCarthy
Recommended Citation
Siddiquee, Mohammad Ashraf. "Machine Learning Techniques for Seismic Data Analysis and Explosion Monitoring." (2022). https://digitalrepository.unm.edu/cs_etds/114