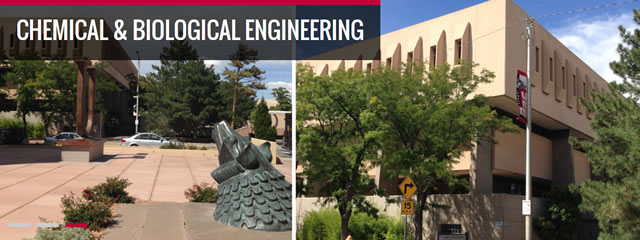
Chemical and Biological Engineering ETDs
Publication Date
Spring 3-31-2021
Abstract
Understanding diffusion of chemical compounds is important for the design and optimization of many chemical engineering and energy processes. Recent modifications to the Darken equation allow for accurate prediction of Maxwell-Stefan (MS) diffusion in mixtures. Still, there are few practical applications due to the requirement of individual self-diffusion constants. A reliable predictive model for self-diffusion constants would be highly valuable when used in conjunction with the modified Darken equation. Here, we show that Machine Learning (ML) can be used to develop generalized models for self-diffusion in Lennard Jones (LJ) systems and real systems of pure solutions. The use of Artificial Neural Networks (ANNs) leads to accurate, generalized predictions for self-diffusion in pure compounds across liquid, gas, and supercritical states. Feature importance assignments also reveal which features most impact diffusion predictions and results are related back to known physical relationships and correlations.
Keywords
Machine Learning, Self-Diffusion, Artificial Neural Network
Document Type
Thesis
Language
English
Degree Name
Chemical Engineering
Level of Degree
Masters
Department Name
Chemical and Biological Engineering
First Committee Member (Chair)
Fernando H. Garzon
Second Committee Member
Todd M. Alam
Third Committee Member
P. Randall Schunk
Recommended Citation
Allers, Joshua P.. "PREDICTION OF SELF-DIFFUSION CONSTANTS IN MODEL AND REAL SYSTEMS USING MACHINE LEARNING." (2021). https://digitalrepository.unm.edu/cbe_etds/89