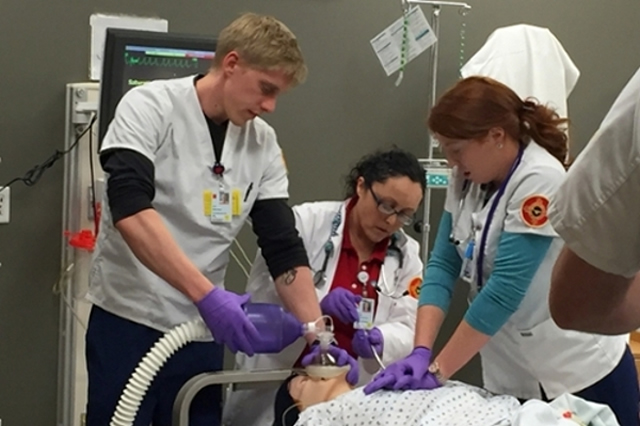
Nursing ETDs
Publication Date
Fall 12-31-2022
Abstract
A problem of classification places adolescents and emerging adults with special health care needs among the most at risk for poor or life-threatening health outcomes. This preliminary proof-of-concept study was conducted to determine if phenotypes of health care transition (HCT) for this vulnerable population could be established. Such phenotypes could support development of future studies that require data classifications as input. Mining of electronic health record data and cluster analysis were implemented to identify phenotypes. Subsequently, a machine learning concept model was developed for predicting acute care and medical condition severity. Three clusters were identified and described (Cluster 1, n = 36,075; Cluster 2, n = 74,438; Cluster 3, n = 6,742). Baseline metrics indicated that the machine learning models performed better than naïve forecasting (MASE [Model 1, 0.5], [Model 2, 0.7]).
An analysis of state and federal policies using a dynamics framework was then conducted to identify policy interdependencies, including policy actors, institutional structures, and intergovernmental agreements relevant to HCT. Policy components identified were in the categories of insurance/payer implications and innovations, HCT surveillance, HCT services and workforce, and digital innovations and interoperability. These included waiver authorities under the Social Security Act; policy institutions and actors associated with HCT surveillance and workforce policy, including the US Health Resources and Services Administration (HRSA) and the Centers for Medicare and Medicaid Services (CMS); and policy institutions and actors associated with data innovation and interoperability, including CMS and its Interoperability and Patient Access Rule (2020).
Insights from the policy analysis and the HCT phenotype study, including cluster demographics data, were applied in development of recommendations for policy collaboratives and policy research. These include the advancement of HCT surveillance; establishment of an HCT policy collaborative focused on leveraging improvements and innovations in compliance with the CMS Interoperability and Patient Access Rule (2020) and Medicaid waiver authorities; development of a recommendation to CMS to incentivize bonus payments to providers within a Health Professional Shortage Area (HPSA) in Census Divisions 6, 7, and 8, who maintain care of patients with special health care needs after age 18 and show evidence of providing regular preventative and/or specialty care; and promotion of digital health policy that complies with CMS Interoperability and Patient Access Rule (2020) and extends and enhances telehealth, data capture, and data interoperability for people with special health care needs. These recommendations are intended to support HCT classification and standardization, advance HCT services and interoperability, and improve HCT outcomes for adolescents and emerging adults with special health care needs.
Degree Name
Nursing
Level of Degree
Doctoral
Department Name
College of Nursing
First Committee Member (Chair)
Christine E. Kasper, PhD, RN, FAAN, FACSM
Second Committee Member
Barbara I. Damron, PhD, RN, FAAN
Third Committee Member
Antonia M. Villarruel, PhD, RN, FAAN
Fourth Committee Member
Patrick Finley, MS
Keywords
data science, health care transition, health policy, machine learning, phenotype, proof-of-concept
Language
English
Document Type
Dissertation
Recommended Citation
Turk, LisaMarie. "APPLYING DATA SCIENCE AND MACHINE LEARNING TO UNDERSTAND HEALTH CARE TRANSITION FOR ADOLESCENTS AND EMERGING ADULTS WITH SPECIAL HEALTH CARE NEEDS." (2022). https://digitalrepository.unm.edu/nurs_etds/59
Included in
Bioinformatics Commons, Data Science Commons, Models and Methods Commons, Nursing Commons