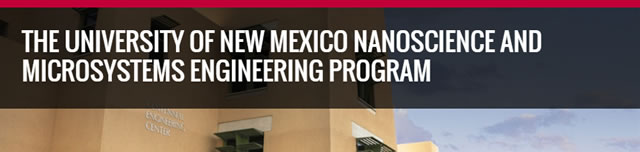
Nanoscience and Microsystems ETDs
Publication Date
2-2-2016
Abstract
The allergic response is produced by the release of immune mediators by mast cells and basophils. This process, in turn, is initiated by the aggregation of antigens and IgE-FcεRI antibody-receptor complexes. Computational modeling of antibody- antigen aggregate formation as well as the size and structure of these aggregates is an important tool for greater understanding of the allergic response. In addition, the incorporation of molecular geometry into aggregation models can more accurately capture details of the aggregation process, and may lead to insights into how geom- etry affects aggregate formation. However, it is challenging to simulate aggregation due to the computational cost of simulating large molecules. Methods to geometri- cally model antibody aggregation inspired by rigid body robotic motion simulations have previously been developed; however, high computational cost mandates that the resolution of the 3D molecular models be reduced, which affects the results of the simulation. Rule-based modeling can be used to model aggregation with low computational cost, but traditional rule-based modeling approaches do not include details of molecular geometry. In this work, we propose a novel implementation of rule-based modeling that encodes details of molecular geometry into the rules and the binding rate constant associated with each rule. We demonstrate how the set of rules is constructed accord- ing to the curvature of the molecule. We then perform a study of antigen-antibody aggregation using our proposed method combined with a previously developed 3D rigid-body Monte Carlo simulation. We first simulate the binding of IgE antibodies bound to cell surface receptors FcεRI to various binding regions of the allergen Pen a 1 using the aforementioned Monte Carlo simulation, and we analyze the distribution of the sizes of the aggregates that form during the simulation. Then, using our novel rule-based approach, we optimize a rule-based model according to the geometry of the Pen a 1 molecule and the data from the Monte Carlo simulation. In particular, we use the distances between the binding regions of the Pen a 1 molecule to optimize the rules and associated binding rate constants. The optimized rule-based models provide information about the average steric hindrance between binding regions and the probability that IgE-FcεRI receptor complexes will bind to these regions. In ad- dition, the optimized rule-based models provide a means of quantifying the variation in aggregate size distribution that results from differences in molecular geometry. We perform this procedure for seven resolutions and three molecular conforma- tions of Pen a 1. We then analyze the impact of resolution and conformation on the aggregate size distribution and on the optimal rule-based model. In addition, we develop a predictive model by first fixing the rule set and varying only the binding rate constant for each resolution, and then fitting the resulting data to a function. This model is intended to enable the prediction of the aggregate size distribution for higher resolutions while requiring only data for lower resolution Monte Carlo models, thus enhancing computational efficiency. Finally, we use a simple rule-based model to fit experimental cell degranulation data for various concentrations of the shrimp allergen Pen a 1 and the IgE antibody.
Keywords
Antigen-antibody reactions--Computer simulation., Molecules--Models., Monte Carlo method., Rule-based programming., Binding sites (Biochemistry)
Sponsors
National Institutes of Health
Document Type
Thesis
Language
English
Degree Name
Nanoscience and Microsystems
Level of Degree
Masters
Department Name
Nanoscience and Microsystems
First Committee Member (Chair)
Tapia, Lydia
Second Committee Member
Estrada, Trilce
Third Committee Member
Hlavacek, William
Fourth Committee Member
Jacobson, Bruna
Recommended Citation
Hoard, Brittany. "Modeling steric effects in antibody aggregation using rule-based methods." (2016). https://digitalrepository.unm.edu/nsms_etds/25