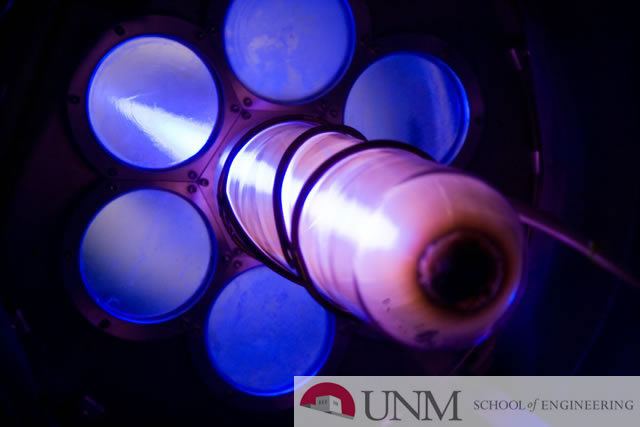
Mechanical Engineering ETDs
Publication Date
6-24-2015
Abstract
The commercial building sector consumed about 20% of the total primary energy in the U.S. in 2008. A significant yet avoidable portion of the energy consumption is due to inefficient system operations. The inefficiencies can be attributed to degrading HVAC sub-systems, and undetected abnormal conditions. Recognition and remediation of these conditions through advanced data analytics can reduce energy consumption by 5% to 20%. This could save about $9 billion in utility costs in the U.S. alone. Modern buildings are constantly sending messages in the form of sensor data. However, this data is only as good as the system that collects it. Therefore, the present work explores fault detection and diagnostics (FDD) of an HVAC sub-system, in particular an air handling unit (AHU), through the evaluation of various methods. The detection methods include a controls alarm threshold, rule-based expressions, regression, one-class support vector machine (SVM), back-propagation, adaptive resonance theory (ART), and lateral priming adaptive resonance theory (LAPART). The diagnosis of AHU faults were performed using a multi-class SVM and LAPART algorithms. The results from the fault detection experiments were reviewed based on the two-class classification where the number of false positives and false negatives where compared. The diagnostic results were evaluated based on the comparison of precision and probability of detection values.
Keywords
air handling unit, artificial neural networks, adaptive resonance theory, laterally primed adaptive resonance theory, support vector machines, fault detection, fault diagnostics, HVAC, machine learning
Degree Name
Mechanical Engineering
Level of Degree
Doctoral
Department Name
Mechanical Engineering
First Committee Member (Chair)
Caudell, Thomas
Second Committee Member
Tapia, Lydia
Third Committee Member
Sorrentino, Francesco
Sponsors
Yearout Mechanical Inc.
Document Type
Dissertation
Language
English
Recommended Citation
Jones, Christian Birk. "Fault detection and diagnostics of an HVAC sub-system using adaptive resonance theory neural networks." (2015). https://digitalrepository.unm.edu/me_etds/28