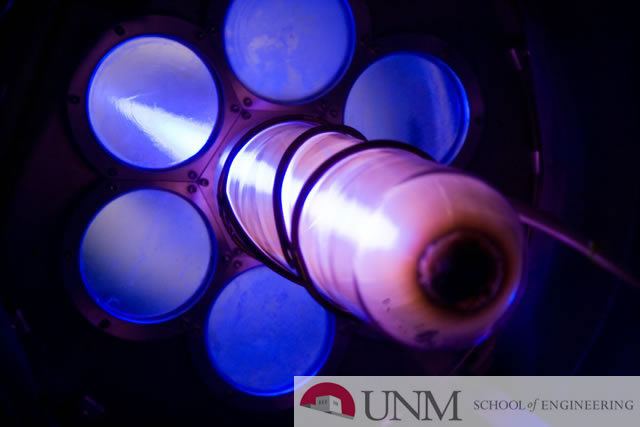
Mechanical Engineering ETDs
Publication Date
Spring 4-8-2019
Abstract
Converting energy from the currents found within tidal channels, open ocean, rivers, and canals is a promising yet untapped source of renewable energy. In order to permit current energy converters for installation in the environment, the CECs must be shown to non-negatively impact the environment. While developing these model increased utility may be gained if researchers may optimize mechanical power while constraining environmental effects. Surrogate models have garnered interest as optimization tools because they maximize the utility of expensive information by building predictive models in place of computational or experimentally expensive model runs. Marine hydrokinetic current energy converters require large-domain simulations to estimate array efficiencies and environmental impacts. Meso-scale models typically represent turbines as actuator discs that act as momentum sinks and sources of turbulence. An OpenFOAM model was developed where actuator-disc $k$-$\epsilon$ turbulence was characterized using an approach developed for flows through vegetative canopies. Turbine-wake data from laboratory flume experiments collected at two influent turbulence intensities were used to calibrate parameters in the turbulence-source terms in the $k$-$\epsilon$ equations. Parameter influences on longitudinal wake profiles were estimated using Gaussian-process regression with subsequent optimization achieving results within 3\% of those obtained using the full model representation, but for as low as 27\% of the computational cost (far fewer model runs). This framework facilitates more efficient parameterization of the turbulence-source equations using turbine-wake data.
Keywords
metamodel, current energy converters, actuator disc, Gaussian process regression, optimization
Degree Name
Mechanical Engineering
Level of Degree
Masters
Department Name
Mechanical Engineering
First Committee Member (Chair)
Sang Lee
Second Committee Member
Humberto III Silva
Third Committee Member
Peter Vorobieff
Fourth Committee Member
Jack CP Su
Document Type
Thesis
Language
English
Recommended Citation
Olson, Sterling. "Gaussian Process Regression applied to Marine Energy Turbulent Source Tuning via Metamodel Machine Learning Optimization." (2019). https://digitalrepository.unm.edu/me_etds/165