Branch Mathematics and Statistics Faculty and Staff Publications
Document Type
Article
Publication Date
2024
Abstract
Graph Neural Networks (GNNs) have emerged as a powerful tool for node representation learning within graph structures. However, designing a robust GNN architecture for node classification remains a challenge. This study introduces an efficient and straightforward Residual Attention Augmentation GNN (RAA-GNN) model, which incorporates an attention mechanism with skip connections to discerningly weigh node features and overcome the over-smoothing problem of GNNs. Additionally, a novel MixUp data augmentation method was developed to improve model training. The proposed approach was rigorously evaluated on various node classification benchmarks, encompassing both social and citation networks. The proposed method outperformed state-of-the-art techniques by achieving up to 1% accuracy improvement. Furthermore, when applied to the novel Twitch social network dataset, the proposed model yielded remarkably promising results. These findings provide valuable insights for researchers and practitioners working with graph-structured data.
Publication Title
Engineering, Technology & Applied Science Research
Volume
14
Issue
2
First Page
13238
Last Page
13242
DOI
https://doi.org/10.48084/etasr.6844
Language (ISO)
English
Keywords
graph neural networks, node classification, over-smoothing, citation and social networks, mixup data augmentation
Recommended Citation
Abbas, Muhammad Affan; Waqar Ali; Florentin Smarandache; Sultan S. Alshamrani; Muhammad Ahsan Raza; Abdullah Alshehri; and Mubashir Ali.
"Residual Attention Augmentation Graph Neural Network for Improved Node Classification Residual Attention Augmentation Graph Neural Network for Improved Node Classification."
Engineering, Technology & Applied Science Research
Creative Commons License
This work is licensed under a Creative Commons Attribution 4.0 International License.
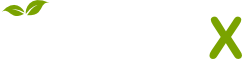
- Citations
- Citation Indexes: 1
- Usage
- Downloads: 21
- Abstract Views: 1
- Captures
- Readers: 5
- Mentions
- News Mentions: 2