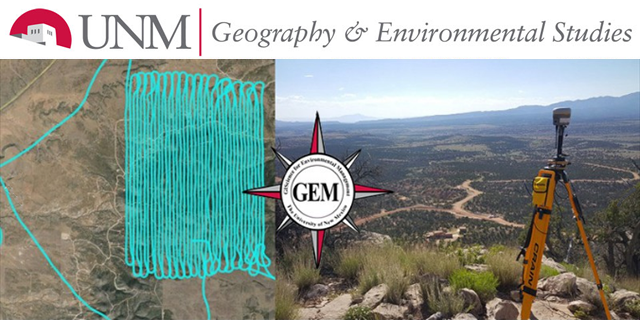
Geography ETDs
Publication Date
Fall 12-15-2024
Abstract
Aerial imaging for wildlife monitoring is entering a rapid phase of methodological development with the advent of computer vision methods enabling efficient and accurate automated image processing in a “remote sensing / machine learning” workflow. In this dissertation, I present a framework for assessing the validity of inputs and outputs of convolutional neural networks trained to detect and classify wildlife in aerial imagery. I examined bias in the processes of training data annotation, machine learning model optimization, and the aerial survey itself, and offered recommendations on structuring aerial surveys. This work was conducted in the context of assisting the US Fish and Wildlife Service in developing an automated workflow for conducting annual waterfowl counts at wildlife refuges in New Mexico, and provides a model of how interdisciplinary methods can aid wildlife monitoring via the integration of participatory science, remote sensing/GIScience, and computer vision.
Degree Name
Geography
Department Name
Geography
Level of Degree
Doctoral
First Committee Member (Chair)
Christopher Lippitt
Second Committee Member
Michaela Buenemann
Third Committee Member
Liping Yang
Fourth Committee Member
Steven Sesnie
Document Type
Dissertation
Project Sponsors
US Fish and Wildlife Service
Keywords
computer vision, UAS, deep learning, waterfowl, bias assessment, wildlife survey
Recommended Citation
Converse, Rowan L.. "Validating a Remote Sensing / Machine Learning Framework for Wildlife Monitoring." (2024). https://digitalrepository.unm.edu/geog_etds/80