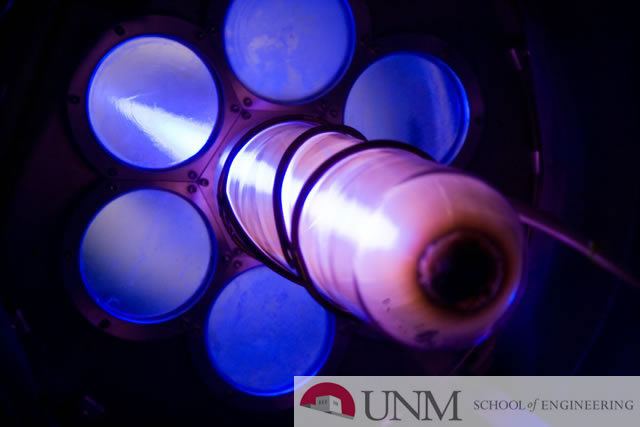
Electrical and Computer Engineering ETDs
Publication Date
Fall 12-15-2024
Abstract
Source detection and automatic modulation classification are two of the most important steps in any array processing task. In this research a novel deep learning model referred to as RadioNet, is proposed that is focused on solving both these problems by reformulating them as a multi-label classification problem. Traditional approaches to both these topics face challenges in scenarios with noise, interference, fewer number of snapshots, and high number of sources. The limitations of the conventional models are investigated and overcome by the proposed solution. RadioNet is also compared with other existing state-of-the-art machine learning based solutions. The introduced model is also implemented and validated using over-the-air measurements. Finally, the proposed framework is shown to perform both source detection and modulation classification at the same time for a limited number of sources and modulation schemes. Thus, this way the effectiveness of the proposed methodologies is evaluated and demonstrated.
Keywords
antenna array processing, source detection, modulation classification, deep learning
Document Type
Dissertation
Language
English
Degree Name
Electrical Engineering
Level of Degree
Doctoral
Department Name
Electrical and Computer Engineering
First Committee Member (Chair)
Christos Christodoulou
Second Committee Member
Mark Gilmore
Third Committee Member
Jehanzeb Chaudhry
Third Advisor
Arjun Gupta
Recommended Citation
Vijayamohanan, Jayakrishnan. "Source detection and automatic modulation classification for modern antenna array processing." (2024). https://digitalrepository.unm.edu/ece_etds/697