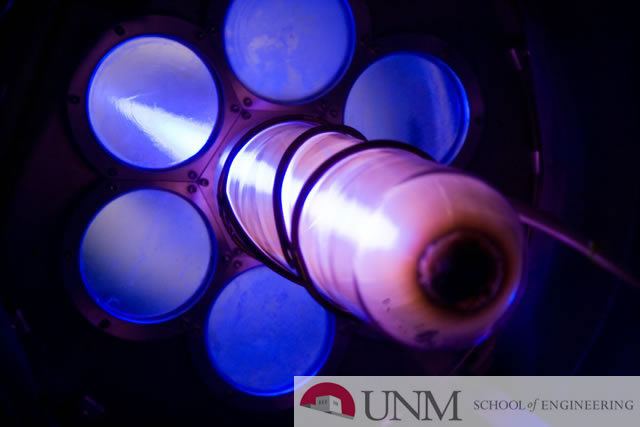
Electrical and Computer Engineering ETDs
Publication Date
Summer 7-25-2024
Abstract
Community-driven energy initiatives have become crucial for effective energy management, particularly in trading and management. The rise of Distributed Energy Resources in smart grids demands a redesign of traditional Demand Response Management (DRM) models to account for prosumers' dynamic behavior in energy markets. Decentralization, including peer-to-peer (P2P) energy trading, is vital for resilience and sustainability. This thesis introduces two coalitional DRM models: one based on hedonic community formation games, and the other combining matching theory with coalition games. These models empower prosumers to autonomously select energy trading communities using partially available data. To optimize energy consumption, two additional models are proposed—one using log-linear reinforcement learning, and the other a distributed game-theoretic approach. Furthermore, a non-cooperative pricing model for P2P markets and a reinforcement learning mechanism for EV energy trading are developed. Simulation results validate the effectiveness and scalability of these models, demonstrating their superior performance.
Keywords
Game Theory, Reinforcement Learning, Demand Response Management, Distributed Energy Resources, Peer-to-Peer Trading
Document Type
Dissertation
Language
English
Degree Name
Computer Engineering
Level of Degree
Doctoral
Department Name
Electrical and Computer Engineering
First Committee Member (Chair)
Eirini Eleni Tsiropoulou
Second Committee Member
Jim Plusquellic
Third Committee Member
Aris Leivadeas
Fourth Committee Member
Payman Zarkesh-Ha
Recommended Citation
Kemp, Nicholas. "Distributed Energy Trading Models Utilizing Reinforcement Learning and Game-Theoretic Based Approaches in Smart Grids." (2024). https://digitalrepository.unm.edu/ece_etds/660
Comments
Removed blank page between Approval page and Title page; added "UNM" to previous degrees after degree name and before year on both title page and Abstract page; fixed spacing between previous degrees; edited abstract to be <150 words.