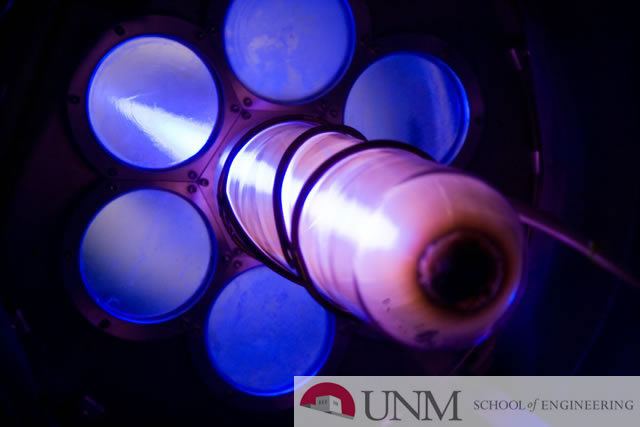
Electrical and Computer Engineering ETDs
Publication Date
Fall 11-30-2023
Abstract
These days large volumes of data can be recorded and manipulated with relative ease. If valuable information can be extracted from them, these vast amounts of data can be a rich resource not just for the digital economy but also for scientific discovery and development of technology. When it comes to deriving valuable information from data, Machine Learning (ML) emerges as the key solution. To unlock the potential benefits of ML to science and technology, extensive research is needed to explore what algorithms are suitable and how they can be applied.
To shine light on various ways that ML can be impactful to engineering workflows, fields of computational EM and antenna design are chosen and efforts are focused on answering three fundamental questions: (1) Is it possible to obtain predictive models from the available simulation and measurement data? (2) What are the domain-specific machine learning algorithms required to convert various datasets to modeling knowledge? (3) Once the modeling knowledge has been learned, how to seamlessly incorporate it into a data-driven predictive environment?
Keywords
AI, Machine Learning, Computational Electromagnetics, FDTD, Antenna Design
Document Type
Dissertation
Language
English
Degree Name
Electrical Engineering
Level of Degree
Doctoral
Department Name
Electrical and Computer Engineering
First Committee Member (Chair)
Christos Christodoulou
Second Committee Member
Zhen Peng
Third Committee Member
Edl Schamiloglu
Fourth Committee Member
Jehanzeb H. Chaudhry
Recommended Citation
Noakoasteen, Oameed. "AI Assisted Workflows for Computational Electromagnetics and Antenna Design." (2023). https://digitalrepository.unm.edu/ece_etds/628
Included in
Artificial Intelligence and Robotics Commons, Data Science Commons, Electrical and Computer Engineering Commons