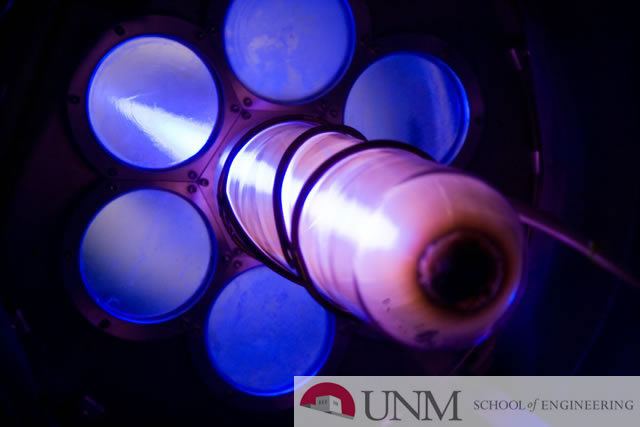
Electrical and Computer Engineering ETDs
Publication Date
Summer 2023
Abstract
Stochastic flaw formation leading to poor print quality is a major obstacle to the utility of directed energy deposition (DED), a laser and metal powder-based additive manufacturing technology for construction and repair of custom metal parts. While melt pool temperature variability is known to be a major factor in flaw formation, control schemes to decrease flaw formation are limited by a lack of physics-based models that fully and accurately describe DED. In this work, a stochastic reachability analysis with a data-driven model based on thermal images of the melt pool was conducted to determine the likelihood of violating melt pool temperature constraints. As validation, the likelihood maps were compared to the true locations of porosities determined from CT scans of the parts. Additionally, a recurrent neural network (RNN) was trained to predict porosity locations using melt pool thermal images without prior assumptions as to the cause of the porosities.
Document Type
Thesis
Language
English
Degree Name
Electrical Engineering
Level of Degree
Masters
Department Name
Electrical and Computer Engineering
First Committee Member (Chair)
Meeko Oishi
Second Committee Member
Rafael Fierro
Third Committee Member
Claus Danielson
Fourth Committee Member
Ganesh Balakrishnan
Recommended Citation
Kaufman, Georgia E.. "Data-driven Porosity Prediction for Directed Energy Deposition." (2023). https://digitalrepository.unm.edu/ece_etds/604