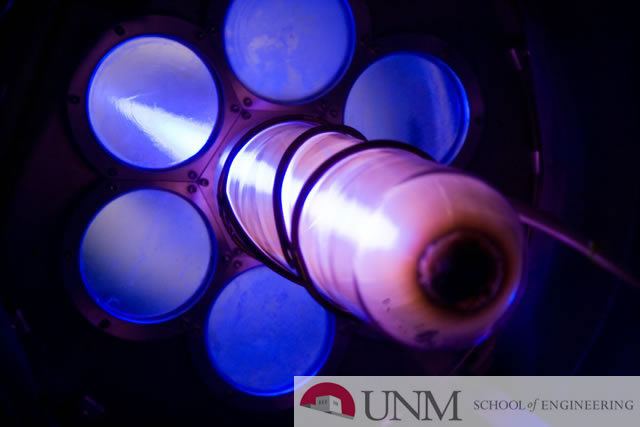
Electrical and Computer Engineering ETDs
Publication Date
Spring 5-13-2023
Abstract
Radionuclide spectroscopic sensor data is analyzed with minimal power consumption through the use of neuromorphic computing architectures. Memristor crossbars are harnessed as the computational substrate in this non-conventional computing platform and integrated with CMOS-based neurons to mimic the computational dynamics observed in the mammalian brain’s visual cortex. Functional prototypes using spiking sparse locally competitive approximations are presented. The architectures are evaluated for classification accuracy and energy efficiency. The proposed systems achieve a 90% true positive accuracy with a high-resolution detector and 86% with a low-resolution detector.
Keywords
RRAM, Neuromorphic Computing, Memristor, Radionuclide Detection
Sponsors
Defense Threat Reduction Agency HDTRA1-18-1-0009
Document Type
Dissertation
Language
English
Degree Name
Electrical Engineering
Level of Degree
Doctoral
Department Name
Electrical and Computer Engineering
First Committee Member (Chair)
Payman Zarkesh-Ha
Second Committee Member
Miguel Ernesto Figueroa Toro
Third Committee Member
Marek Osiński
Fourth Committee Member
Adam Hecht
Fifth Committee Member
Ramiro Jordan
Recommended Citation
Canales Verdial, Jorge Iván. "vi ENERGY-EFFICIENT MEMRISTOR-BASED NEUROMORPHIC COMPUTING CIRCUITS AND SYSTEMS FOR RADIATION DETECTION APPLICATIONS." (2023). https://digitalrepository.unm.edu/ece_etds/597
Included in
Computational Engineering Commons, Computational Neuroscience Commons, Computer and Systems Architecture Commons, Electrical and Electronics Commons, Nanoscience and Nanotechnology Commons, Nuclear Engineering Commons, VLSI and Circuits, Embedded and Hardware Systems Commons