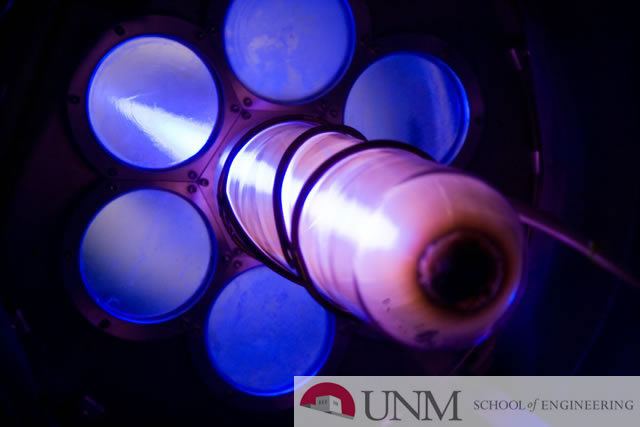
Electrical and Computer Engineering ETDs
Publication Date
Spring 4-13-2023
Abstract
Stochastic disturbances arise in a variety of engineering applications. For tractability, Gaussian disturbances are often assumed. However, this may not always be valid, such as when a disturbance exhibits heavy-tailed or skewed phenomena. As autonomous systems become more ubiquitous, non-Gaussian disturbances will become more common due to the compounding effects of sensing, actuation, and external forces. Despite this, little has been done to develop formal methods that are both computationally efficient and allow for analytical assurances with non-Gaussian disturbances. Addressing convex polytopic set acquisition and non-convex collision avoidance chance constraints with quantile and moment-based reformulations, this dissertation proposes novel stochastic optimal control techniques that are computationally efficient and allow for analytic guarantees with arbitrary disturbances. These reformulations are amenable to optimization techniques while eliminating costly, and frequently intractable, high-dimensional integrals. These conservative reformulations guarantee chance constraint satisfaction and are numerically tractable. I demonstrate these methods with applications to multi-satellite operations.
Keywords
Stochastic optimal control, chance constraints, Control theory, Optimization, Stochastic motion planning
Document Type
Dissertation
Language
English
Degree Name
Electrical Engineering
Level of Degree
Doctoral
Department Name
Electrical and Computer Engineering
First Committee Member (Chair)
Meeko Oishi
Second Committee Member
Rafael Fierro
Third Committee Member
Claus Danielson
Fourth Committee Member
Christopher Petersen
Recommended Citation
Priore, Shawn. "Chance Constrained Stochastic Optimal Control of Discrete Time Linear Stochastic Systems with Applications in Multi-satellite Operations." (2023). https://digitalrepository.unm.edu/ece_etds/594