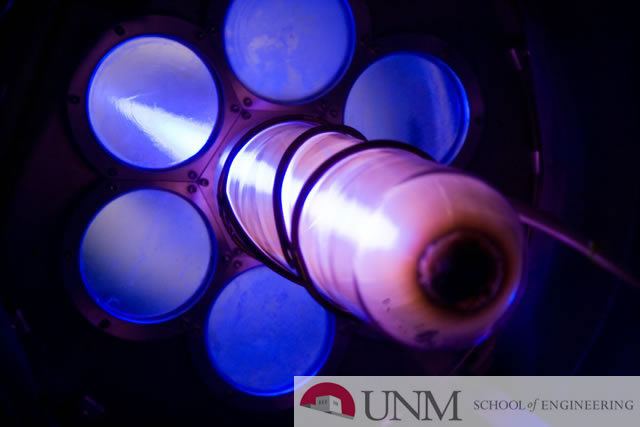
Electrical and Computer Engineering ETDs
Publication Date
Spring 4-13-2023
Abstract
The dissertation develops new methods for assessing student participation in long (>1 hour) classroom videos. First, the dissertation introduces the use of multiple image representations based on raw RGB images and AM-FM components to detect specific student groups. Second, a dynamic scene analysis model is developed for tracking under occlusion and variable camera angles. Third, a motion vector projection system identifies instances of students talking.
The proposed methods are validated using digital videos from the Advancing Out-of-school Learning in Mathematics and Engineering (AOLME) project. The proposed methods are shown to provide better group detection, and better talking detection at 59% accuracy compared to 42% for Temporal Segment Network (TSN) and 45% for Convolutional 3D neural network (C3D), and dynamic scene analysis can track participants at 84.1% accuracy compared to 61.9% for static analysis. The methods are used to create activity maps to visualize and quantify student participation.
Keywords
Group detection, Dynamic participant tracking, AOLME dataset
Document Type
Dissertation
Language
English
Degree Name
Computer Engineering
Level of Degree
Doctoral
Department Name
Electrical and Computer Engineering
First Committee Member (Chair)
Marios Pattichis
Second Committee Member
Ramiro Jordan
Third Committee Member
Xiang Sun
Fourth Committee Member
Sylvia Celedón-Pattichis
Recommended Citation
SHI, WENJING. "Long-term Human Participation Detection Using A Dynamic Scene Analysis Model." (2023). https://digitalrepository.unm.edu/ece_etds/593
Included in
Bilingual, Multilingual, and Multicultural Education Commons, Electrical and Computer Engineering Commons