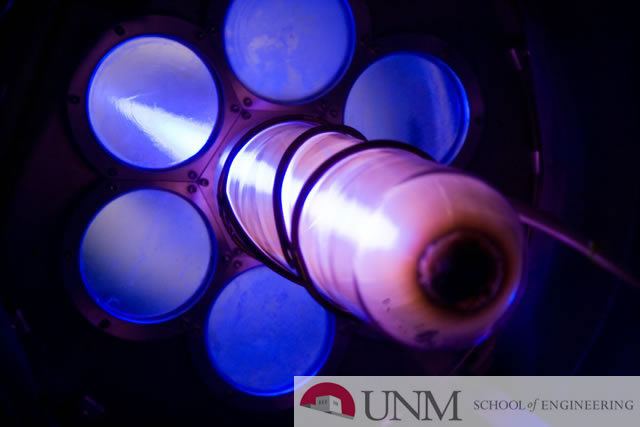
Electrical and Computer Engineering ETDs
Publication Date
Spring 5-13-2023
Abstract
Federated Learning (FL) effectiveness depends, among others, on the quality and quantity of the training data and process realized at the end computing nodes. In this paper, we introduce a novel location-based federated learning model, enabled by a low-cost and fast deployable Reconfigurable Intelligent Surfaces (RIS) - based approach that allows to accurately determine the distributed computing nodes’ positions. Furthermore, in order to train a global model to support different types of smart city applications, while considering two types of servers, offering a prime and common service, respectively, under different costs, the proposed location-based FL model is complemented by an appropriate incentivization mechanism. The latter is based on the theory of Colonel Blotto games and aims at designing the optimal rewards that should be provided to the computing nodes by the servers, in order the former to be properly motivated to exploit a large amount of their raw data towards improving the FL training performance. The outcome of this process depends on the available budget of each server and on each node’s criticality - determined by its position and available data. The performance evaluation of the proposed location-based federated learning model is obtained via modeling and simulation using a real dataset.
Document Type
Thesis
Language
English
Degree Name
Computer Engineering
Level of Degree
Masters
Department Name
Electrical and Computer Engineering
First Committee Member (Chair)
Dr. Eirini Eleni Tsiropoulou
Second Committee Member
Dr. Jim Plusquellic
Third Committee Member
Dr. Iraklis Anagnostopoulos
Recommended Citation
Brown, Ryan Kilpatrick-Morrison. "Incentives to Learn: A Location-based Federated Learning Model." (2023). https://digitalrepository.unm.edu/ece_etds/585