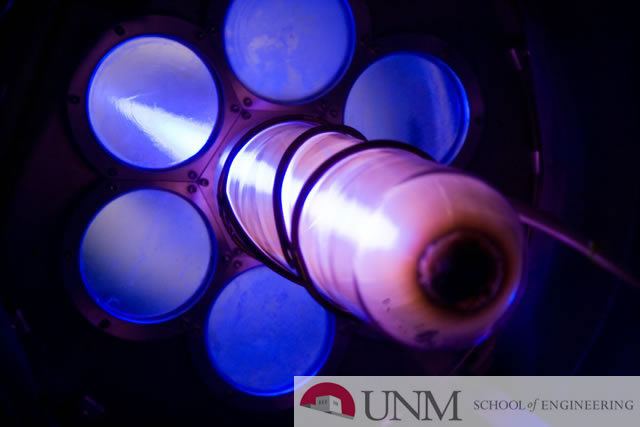
Electrical and Computer Engineering ETDs
Publication Date
Summer 7-30-2021
Abstract
The stochastic fluctuations from Renewable Energy Resources (RER) have a great influence on power quality and off-grid communities. A combination of the different storage systems is accessible for RER generation intermittency and to bring about finest smoothing operating cycle compared to sole Energy Storage System (ESS). Additionally, energy management in Hybrid Energy Storage System (HESS) creates an uncertainty during power smoothing operation. This research materializes, an intelligent mechanism for power smoothing and dispatch with the introduction of hybridized storage that can accommodate the unpredictable behavior of RER under dynamic load. A feed-forward neural network is proposed as a power smoothing model for a RER with supercapacitors (SC) and battery energy storage (BES). The novelty of the research is adopted from the Reinforcement Learning (RL) based Deep Deterministic Policy Gradient (DDPG) approach to control the charge/discharge of HESS by removing severely fluctuating current exchange. The proposed distributed control architecture is developed using the Matlab/Simulink model for analyzing its efficiency in achieving power dispatch, smoothing, and compares to other strategies in the literature in terms of SC coordination with RER and BESS.
Keywords
Hybrid Energy Storage Systems, Neural Network, Power Balancing, Power Dispatch, Power Management, Power Smoothing, Reinforcement Learning
Document Type
Thesis
Language
English
Degree Name
Electrical Engineering
Level of Degree
Masters
Department Name
Electrical and Computer Engineering
First Committee Member (Chair)
Dr. Ali Bidram
Second Committee Member
Dr. Marios Pattichis
Third Committee Member
Dr. Ramiro Jordan
Recommended Citation
Ramalingam, Bhuvaneshwarr. "Machine Learning based Dynamic Power Dispatching and Smoothing using Hybrid Energy Storage System for Renewable Energy Systems." (2021). https://digitalrepository.unm.edu/ece_etds/560