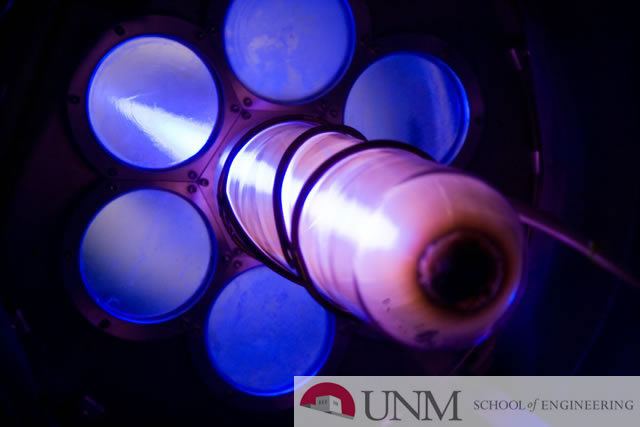
Electrical and Computer Engineering ETDs
Publication Date
Spring 5-2022
Abstract
Speaker identification in noisy audio recordings, specifically those from collaborative learning environments, can be extremely challenging. There is a need to identify individual students talking in small groups from other students talking at the same time. To solve the problem, we assume the use of a single microphone per student group without any access to previous large datasets for training.
This dissertation proposes a method of speaker identification using cross-correlation patterns associated to an array of virtual microphones, centered around the physical microphone. The virtual microphones are simulated by using approximate speaker geometry observed from a video recording. The patterns are constructed based on estimates of the room impulse responses for each virtual microphone. The correlation patterns are then used to identify the speakers. The proposed method is validated with classroom audios and shown to substantially outperform diarization services provided by Google Cloud and Amazon AWS
Keywords
TERMS Speaker Identification, Speaker Diarization, Audio Room Simulation, Virtual Microphone Arrays.
Document Type
Dissertation
Language
English
Degree Name
Electrical Engineering
Level of Degree
Doctoral
Department Name
Electrical and Computer Engineering
First Committee Member (Chair)
Dr. Marios Pattichis Dr. Marios Pattichis
Second Committee Member
Dr. Ramiro Jordan
Third Committee Member
Dr. Sylvia Pattichis
Fourth Committee Member
Dr. Kim Linder
Fifth Committee Member
Dr. Manel Martinez-Ramon
Recommended Citation
Gomez, Antonio. "Speaker Diarization and Identification from Single-Channel Classroom Audio Recording Using Virtual Microphones." (2022). https://digitalrepository.unm.edu/ece_etds/523
Included in
Bilingual, Multilingual, and Multicultural Education Commons, Computational Engineering Commons, Educational Methods Commons, Educational Technology Commons, Science and Mathematics Education Commons, Signal Processing Commons