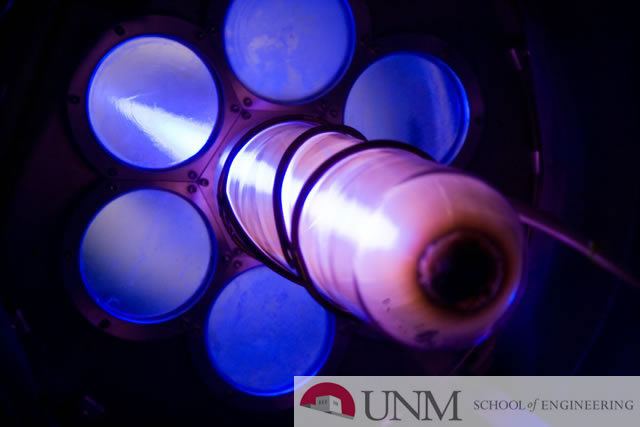
Electrical and Computer Engineering ETDs
Publication Date
Fall 11-1-2019
Abstract
In this thesis, exploiting Fully Autonomous Aerial Systems' (FAAS) and Mobile Edge Computing (MEC) servers' computing capabilities to introduce a novel data offloading framework to support the energy and time-efficient video processing in surveillance systems based on satisfaction games. A surveillance system is introduced consisting of Areas of Interest (AoIs), where a MEC server is associated with each AoI, and a FAAS is flying above the AoIs to support the IP cameras' computing demands. Each IP camera adopts a utility function capturing its Quality of Service (QoS) considering the experienced time and energy overhead to offload and process remotely or locally the data. A non-cooperative game among the cameras is formulated to determine the amount of offloading data to the MEC server and/or the FAAS, and the novel concept of Satisfaction Equilibrium (SE) is introduced where the IP cameras satisfy their minimum QoS prerequisites instead of maximizing their performance by consuming additional system resources. A distributed learning algorithm determines the IP cameras' stable data offloading. Also, a reinforcement learning algorithm indicates the FAAS's movement among the AoIs exploiting the accuracy, timeliness, and certainty of the collected data by the IP cameras per AoI. Detailed numerical and comparative results are presented to show the operation and efficiency of the proposed framework.
Keywords
Surveillance Systems, Mobile Edge Computing, Fully Autonomous Aerial Systems, Reinforcement Learning, Satisfaction Games
Document Type
Thesis
Language
English
Degree Name
Computer Engineering
Level of Degree
Masters
Department Name
Electrical and Computer Engineering
First Committee Member (Chair)
Eirini-Eleni Tsiropoulou
Second Committee Member
Michael Devetsikiotis
Third Committee Member
Jim Plusquellic
Recommended Citation
Torres, Marcos Paul. "Satisfaction-Aware Data Offloading in Surveillance Systems." (2019). https://digitalrepository.unm.edu/ece_etds/477