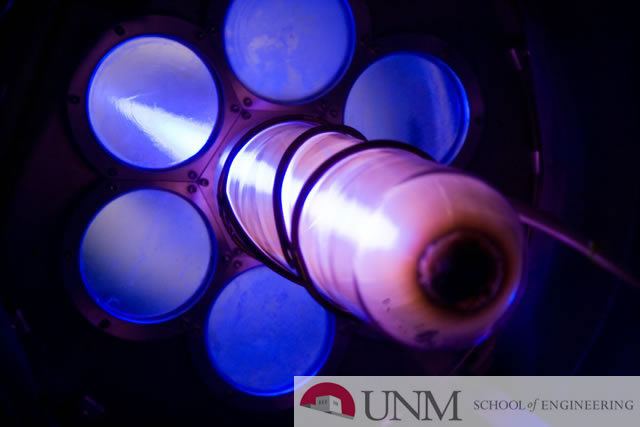
Electrical and Computer Engineering ETDs
Publication Date
Fall 11-15-2018
Abstract
Neuroimaging technologies aim at delineating the highly complex structural and functional organization of the human brain. In recent years, several unimodal as well as multimodal analyses of structural MRI (sMRI) and functional MRI (fMRI) neuroimaging modalities, leveraging advanced signal processing and machine learning based feature extraction algorithms, have opened new avenues in diagnosis of complex brain syndromes and neurocognitive disorders. Generically regarding these neuroimaging modalities as filtered, complimentary insights of brain’s anatomical and functional organization, multimodal data fusion efforts could enable more comprehensive mapping of brain structure and function.
Large scale functional organization of the brain is often studied by viewing the brain as a complex, integrative network composed of spatially distributed, but functionally interacting, sub-networks that continually share and process information. Such whole-brain functional interactions, also referred to as patterns of functional connectivity (FC), are typically examined as levels of synchronous co-activation in the different functional networks of the brain. More recently, there has been a major paradigm shift from measuring the whole-brain FC in an oversimplified, time-averaged manner to additional exploration of time-varying mechanisms to identify the recurring, transient brain configurations or brain states, referred to as time-varying FC state profiles in this dissertation. Notably, prior studies based on time-varying FC approaches have made use of these relatively lower dimensional fMRI features to characterize pathophysiology and have also been reported to relate to demographic characterization, consciousness levels and cognition.
In this dissertation, we corroborate the efficacy of time-varying FC state profiles of the human brain at rest by implementing statistical frameworks to evaluate their robustness and statistical significance through an in-depth, novel evaluation on multiple, independent partitions of a very large rest-fMRI dataset, as well as extensive validation testing on surrogate rest-fMRI datasets. In the following, we present a novel data-driven, blind source separation based multimodal (sMRI-fMRI) data fusion framework that uses the time-varying FC state profiles as features from the fMRI modality to characterize diseased brain conditions and substantiate brain structure-function relationships. Finally, we present a novel data-driven, deep learning based multimodal (sMRI-fMRI) data fusion framework that examines the degree of diagnostic and prognostic performance improvement based on time-varying FC state profiles as features from the fMRI modality. The approaches developed and tested in this dissertation evince high levels of robustness and highlight the utility of time-varying FC state profiles as potential biomarkers to characterize, diagnose and predict diseased brain conditions. As such, the findings in this work argue in favor of the view of FC investigations of the brain that are centered on time-varying FC approaches, and also highlight the benefits of combining multiple neuroimaging data modalities via data fusion.
Keywords
Functional MRI, Functional Connectivity, Structural MRI, Multimodal Data Fusion, Deep Learning, Brain Disease Characterization, Brain Disease Prediction, Schizophrenia, Alzheimer's Disease
Document Type
Dissertation
Language
English
Degree Name
Electrical Engineering
Level of Degree
Doctoral
Department Name
Electrical and Computer Engineering
First Committee Member (Chair)
Dr. Vince D. Calhoun
Second Committee Member
Dr. Ramiro Jordan
Third Committee Member
Dr. Manel Martinez-Ramon
Fourth Committee Member
Dr. Robyn L. Miller
Recommended Citation
Abrol, Anees. "Frameworks to Investigate Robustness and Disease Characterization/Prediction Utility of Time-Varying Functional Connectivity State Profiles of the Human Brain at Rest." (2018). https://digitalrepository.unm.edu/ece_etds/439