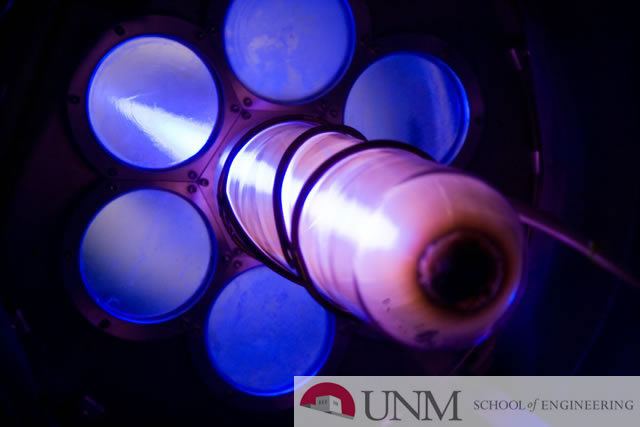
Electrical and Computer Engineering ETDs
Publication Date
Summer 7-31-2017
Abstract
A college student's success depends on many factors including pre-university characteristics and university student support services. Student graduation rates are often used as an objective metric to measure institutional effectiveness. This work studies the impact of such factors on graduation rates, with a particular focus on delay in graduation. In this work, we used feature selection methods to identify a subset of the pre-institutional features with the highest discriminative power. In particular, Forward Selection with Linear Regression, Backward Elimination with Linear Regression, and Lasso Regression were applied. The feature sets were selected in a multivariate fashion. High school GPA, ACT scores, student's high school, financial aid received, and first generation status were found to be important for predicting success. In order to predict delay in graduation, we trained predictive models using Support Vector Machines (SVMs), Gaussian Processes (GPs), and Deep Boltzmann Machines (DBMs) on real student data. The difference in performance among the models is negligible with respect to overall accuracies obtained. Further analysis showed that DBMs outperform SVMs in terms of precision and recall for individual classes. However, the DBM and SVM implementations are computationally inexpensive compared to GPs, given the same resources.
Keywords
Higer Education, Analytics, Machine Learning, Data Mining, Predicitve Analytics, Feature Selection, Graduation Delay, Graduation Rate
Document Type
Thesis
Language
English
Degree Name
Computer Engineering
Level of Degree
Masters
Department Name
Electrical and Computer Engineering
First Committee Member (Chair)
Gregory L Heileman
Second Committee Member
Manel Martinez-Ramon
Third Committee Member
Don R Hush
Fourth Committee Member
Ahmad Slim
Recommended Citation
Ojha, Tushar. "Prediction of Graduation Delay Based on Student Characterisitics and Performance." (2017). https://digitalrepository.unm.edu/ece_etds/355