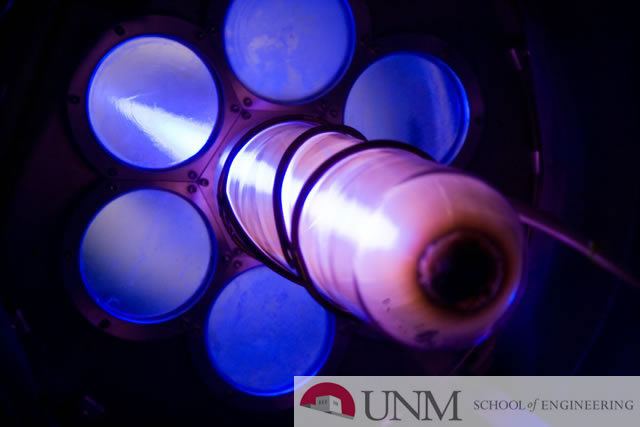
Electrical and Computer Engineering ETDs
Publication Date
Fall 11-15-2016
Abstract
Solar image analysis relies on the detection of coronal holes for predicting disruptions to earth’s magnetic field. The coronal holes act as sources of solar wind that can reach the earth. Thus, coronal holes are used in physical models for predicting the evolution of solar wind and its potential for interfering with the earth’s magnetic field. Due to inherent uncertainties in the physical models, there is a need for a classification system that can be used to select the physical models that best match the observed coronal holes. The physical model classification problem is decomposed into three subproblems. First, the thesis develops a method for coronal hole segmentation. Second, the thesis develops methods for matching coronal holes from different maps. Third, based on the matching results, the thesis develops a physical map classification system. A level-set segmentation method is used for detecting coronal holes that are observed in extreme ultra-violet images (EUVI) and magnetic field images. For validating the segmentation approach, two independent manual segmentations were combined to produce 46 consensus maps. Overall, the level-set segmentation ap- proach produces significant improvements over current approaches. Coronal hole matching is broken into two steps. First, an automated method is used to combine coronal holes into clusters. Second, a Linear Program formulation is used for matching the clusters. The results are validated using manual clustering and matching. Compared to manual matching, the automated matching method gave more than 85 percent accuracy. Physical map classification is based on coronal hole matching between the physical maps and (i) the consensus maps (semi-automated), or (ii) the segmented maps (fully-automated). Based on the matching results, the system uses area differences, shortest distances between matched clusters, number and areas of new and missing coronal hole clusters to classify each map. The results indicate that the automated segmentation and classification system performs better than individual humans.
Keywords
soalr, synoptic, ivpcl, image analysis, magnetic photomap, level sets, subjective analysis
Document Type
Thesis
Language
English
Degree Name
Computer Engineering
Level of Degree
Masters
Department Name
Electrical and Computer Engineering
First Committee Member (Chair)
Prof. Marios Pattichis
Second Committee Member
Prof. Mark Gilmore
Third Committee Member
Dr. Nick Charles Arge
Recommended Citation
Jatla, Venkatesh. "Automatic Segmentation of Coronal Holes in Solar Images and Solar Prediction Map Classification." (2016). https://digitalrepository.unm.edu/ece_etds/301