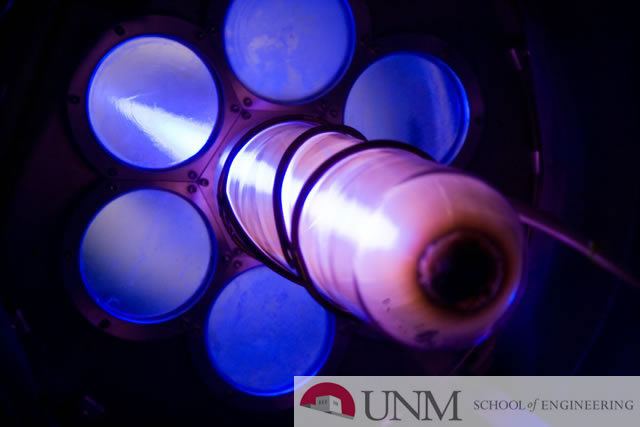
Electrical and Computer Engineering ETDs
Publication Date
8-25-2016
Abstract
The integration of Renewable Energy (RE) into Power Systems brings new challenges to the Smart Grids (SG) technologies. The generation output from renewable sources generally depends on the atmospheric conditions. This fact causes intermittences on the power output from renewable source, and hence the power quality of the grid is directly affected by atmospheric phenomena. The increasing advances on technologies for energy storage open a track to the Energy Management (EM). Therefore, the power output from a renewable source can be stored or dispatched in a particular time-instant in order to meet the demand. Scheduling Demand Respond (DR) action on the grid, can optimize the dispatch by reducing over generated energy wastage. The difficulty now is to ensure the availability of energy to supply into the grid by forecasting the Global Solar Radiation (GSR) on a localization where a Photovoltaic (PV) system is connected. This thesis tries to address the issue using Machine Learning (ML) techniques. This eases the generation scheduling task. The work developed on this thesis is focused on exploring ML techniques to hourly forecast GSR and optimize the dispatch of energy on a SG. The experiments present results for different configuration of Deep Learning and Gaussian Processes for GSR time-series regression, aiming to discuss the advantages of using hybrid methods on the context of SG.
Document Type
Thesis
Language
English
Degree Name
Electrical Engineering
Level of Degree
Masters
Department Name
Electrical and Computer Engineering
First Committee Member (Chair)
Jordan, Ramiro
Second Committee Member
Christodoulou, Christos
Recommended Citation
Terren-Serrano, Guillermo. "Machine Learning Approach to Forecast Global Solar Radiation Time Series." (2016). https://digitalrepository.unm.edu/ece_etds/249