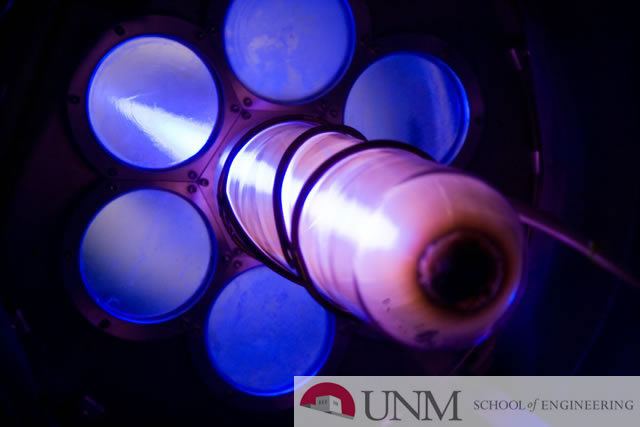
Electrical and Computer Engineering ETDs
Publication Date
6-28-2010
Abstract
The main thrust of this dissertation is to develop, prototype and test new customized algorithms for infrared spectral imaging and remote sensing of terrestrial features and objects, with particular focus on a general class of sensors with noisy and overlapping spectral bands. While the principal driver of this dissertation is the bias-tunable quantum-dot-in-a-well (DWELL) sensor, developed at the Center for High Technology Materials (CHTM), the scope of the proposed algorithms is broad and extends to traditional sensors with fixed bands. The algorithms formulated in this work also represent a step towards enabling future compressive sensing capabilities based on bias tunable detectors such as the DWELL imager. The key contributions of this dissertation span three distinct types of algorithms for multispectral (MS) and hyperspectral (HS) imagery. The first algorithm is the Canonical Correlation Feature Selection (CCFS), which utilizes the spectral content of the data to form a weighted linear superposition of the bias-tunable DWELL bands in order to achieve algorithmic spectral matching in the presence of noise for the purpose of feature selection and classification. Mathematically, the CCFS can be interpreted as a customized projection algorithm, which minimizes a generalized distance between the spectrum of an object and the linear space spanned by the filter\'s bands, while maximizing the signal-to-noise ratio. The second algorithm extends the CCFS to a spatio-spectral feature selection framework. In this framework the spatial content of the HS imagery is used to enhance the canonical spectral features exposed by the CCFS algorithm by applying a sequence of spatial masks to the canonical features. The resulting "stacked" hyperpixels combine spatial and spectral features that have been obtained independently from each other. Consequently, the extension of the CCFS rests on the assumption of spatial-spectral separability of the hyperspectral image, i.e., that the spatial content of a hyperspectral image is independent of the spectral bands, but can be used to enhance the latter. The third algorithm is a joint spatio-spectral algorithm for MS and HS image segmentation, which integrates the spectral information into the spatial feature extraction process in order to achieve simultaneous spatio-spectral feature selection. Unlike the second algorithm, this algorithm does not assume spatial-spectral separability of the hyperspectral image. Instead, it utilizes the concept of spectral ratio contrast to define edge signatures using bands that maximize the spectral contrast between any two materials based on their spatio-spectral signatures. The edge signatures are then fused with a spatial mask to obtain a three-dimensional spatio-spectral mask in which the third (spectral) dimension of each pixel can be independently chosen. This dissertation also provides a thorough and systematic validation and testing of the three algorithms using laboratory data and real infrared imagery from the DWELL Focal Plane Array (FPA) and the Airborne Hyperspectral Imager (AHI). Our results include supervised classification and spectral unmixing and abundance estimation using AHI hyperspectral imagery, rock classification study using laboratory and DWELL FPA imagery, and HS and MS edge detection using the AHI and the DWELL FPA imagery.'
Keywords
Remote sensing images--Data processing., Multispectral photography--Data processing., Infrared imaging--Data processing., Image processing--Digital techniques., Pattern recognition systems., Computer algorithms.
Sponsors
National Science Foundation under awards IIS-0434102 and ECS-401154; additional support was provided by the National Consortium for MASINT Research through a Partnership Project led by the Los Alamos National Laboratory.
Document Type
Dissertation
Language
English
Degree Name
Electrical Engineering
Level of Degree
Doctoral
Department Name
Electrical and Computer Engineering
First Committee Member (Chair)
Caudell, Thomas
Second Committee Member
Krishna, Sanjay
Third Committee Member
Prasad, Sudhakar
Fourth Committee Member
Santhanam, Bal
Recommended Citation
Paskaleva, Biliana. "Algorithms for spectral and spatio-spectral feature selection and classification for tunable sensors : theory and application." (2010). https://digitalrepository.unm.edu/ece_etds/200