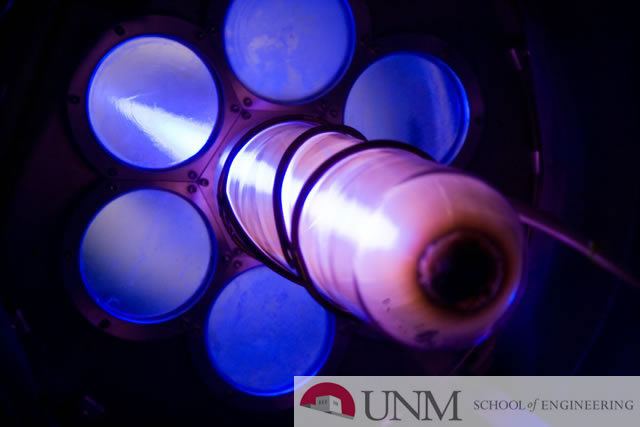
Electrical and Computer Engineering ETDs
Publication Date
1-28-2015
Abstract
In this dissertation, a hierarchical interactive architecture for the future smart grid is proposed. This hierarchical architecture consists of different layers ranging from the households, microgrid controller level, feeder level and substation level. The proposed smart grid architecture is scalable while allowing for sufficient resource pooling, because in each layer the power generation and consumption sides interact in a similar manner. Therefore, we develop an abstract Grid model with distributed energy resources (DER) and storage facilities. A comprehensive real time interactive scheme is proposed for the abstract Grid model, which addresses several important topics: (1) load prediction and uncertainty modeling, (2) demand response (DR), (3) stochastic tracking control of the conventional generation in the presence of DER's (both renewable energy and plug-in hybrid electric vehicle (PHEV)) and (4) machine learning aided decision making for smart-homes. In the first part, a series of linear prediction models are presented for the load prediction purposes, including standard autoregressive (AR) process and time varying autoregressive (TVAR) process, according to different assumptions on the stationarity of customer load profile: piecewise stationarity, local stationarity and cyclo-stationarity. Two important issues in AR/TVAR models are addressed: determining the order of AR/TVAR models and calculating the AR/TVAR coefficients. The partial autocorrelation function (PACF) is analyzed to determine the model order and the minimum mean squared error (MMSE) estimator is adopted to derive the AR/TVAR coefficients, which leads to the Yule-Walker type of equations. In the second part, a DR scheduling scheme based on the Utility cost minimization with different customer clustering sizes. A convex optimization problem is formulated and the optimal demand response profile is in the form of a two-dimensional water-filling solution either with flat water levels or different water levels for different customers. A trade-off strategy which attempts to balance the competing objectives (centralized and distributed) is also provided based on the Price of Anarchy (PoA) analysis. In the third part, two stochastic tracking schemes are proposed to balance the power generation and consumption: (1) reference dynamics-based tracking and (2) reference statistics-based tracking. The proposed optimal tracking control schemes are further generalized by considering the realistic scenario with asynchronous net load demand signals from different customers. Based on the separation principle in reference prediction and tracking design, we propose both centralized and distributed reference prediction schemes based on Kalman filtering technique. In the forth part, with the hierarchical architecture well developed, the smart-home decision making problem is addressed by combining solutions to two subproblems: (1) a hidden mode Markov decision process (HM-MDP) model based centralized sequential decision making at the microgrid controller to maximize an accumulated reward of the whole microgrid and (2) distributed auctioning game design among all smart-homes within the microgrid to coordinate their interactions based on the optimal energy decisions obtained in the centralized sub-problem.
Keywords
Smart Grid, Machine Learning, Adaptive Tracking Control, Stochastic Signal Processing, Game Theory, Optimization
Document Type
Dissertation
Language
English
Degree Name
Electrical Engineering
Level of Degree
Doctoral
Department Name
Electrical and Computer Engineering
First Committee Member (Chair)
Abdallah, Chaouki
Second Committee Member
Graham, Edward
Third Committee Member
Sorrentino, Francesco
Recommended Citation
Li, Ding. "Machine Learning Aided Decision Making and Adaptive Stochastic Control in a Hierarchical Interactive Smart Grid." (2015). https://digitalrepository.unm.edu/ece_etds/160