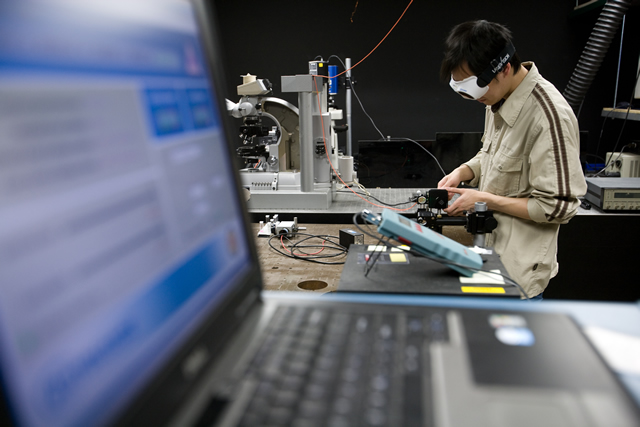
Computer Science ETDs
Publication Date
Summer 7-14-2018
Abstract
In a time when data is being constantly generated by phones, vehicles, sensor net- works, social media, etc. detecting anomalies with in the data can be very crucial. In cases where we know little prior knowledge about the data, it becomes difficult to extract uncertainty about our results. In this thesis, we will propose a framework in which we can extract uncertainty distributions from data-driven modeling prob- lems. We will show some concrete examples of how to apply framework and provide some insight into what the uncertainty distributions are telling us using High Density Regions (HDRs).
Language
English
Keywords
Uncertainty Quantification; High Density Regions; Time Series
Document Type
Dissertation
Degree Name
Computer Science
Level of Degree
Doctoral
Department Name
Department of Computer Science
First Committee Member (Chair)
Trilce Estrada
Second Committee Member
Patrick Bridges
Third Committee Member
Shuang Luan
Fourth Committee Member
David Stracuzzi
Recommended Citation
Peterson, Matthew Gregor. "Data-Driven Uncertainty Quantification Interpretation with High Density Regions." (2018). https://digitalrepository.unm.edu/cs_etds/98