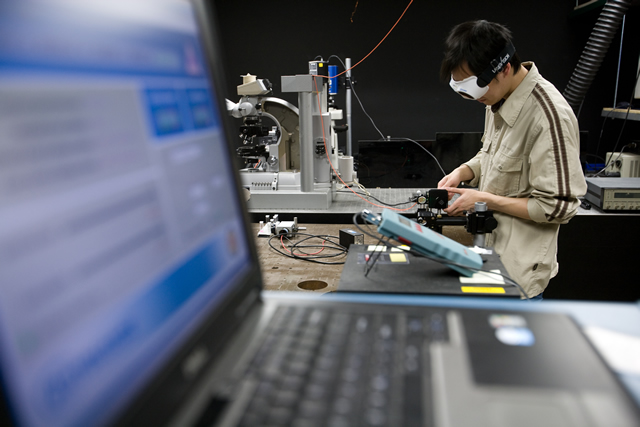
Computer Science ETDs
Publication Date
Fall 11-14-2016
Abstract
Neuroimaging is a growing domain of research, with advances in machine learning having tremendous potential to expand understanding in neuroscience and improve public health. Deep neural networks have recently and rapidly achieved historic success in numerous domains, and as a consequence have completely redefined the landscape of automated learners, giving promise of significant advances in numerous domains of research. Despite recent advances and advantages over traditional machine learning methods, deep neural networks have yet to have permeated significantly into neuroscience studies, particularly as a tool for discovery. This dissertation presents well-established and novel tools for unsupervised learning which aid in feature discovery, with relevant applications to neuroimaging. Through our works within, this dissertation presents strong evidence that deep learning is a viable and important tool for neuroimaging studies.
Language
English
Keywords
Deep learning, neuroimaging, machine learning, variational inference, graphical models
Document Type
Dissertation
Degree Name
Computer Science
Level of Degree
Doctoral
Department Name
Department of Computer Science
First Committee Member (Chair)
Trilce Estrada
Second Committee Member
Vince Calhoun
Third Committee Member
Sergey Plis
Fourth Committee Member
Kyunghyun Cho
Fifth Committee Member
Ruslan Salakhutdinov
Recommended Citation
Hjelm, Rex Devon. "Towards Deeper Understanding in Neuroimaging." (2016). https://digitalrepository.unm.edu/cs_etds/80
Included in
Artificial Intelligence and Robotics Commons, Neuroscience and Neurobiology Commons, Statistical Models Commons