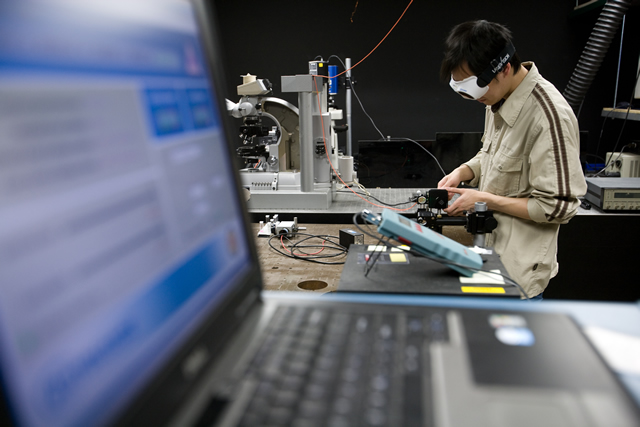
Computer Science ETDs
Publication Date
Spring 5-11-2024
Abstract
Time series data mining and learning serve as a cornerstone across various domains, including finance, healthcare, and science. Recent advancements in network and sensor technologies have ignited an increasing interest in real-time temporal data mining and learning techniques. Various tasks benefit from these techniques, such as environmental monitoring, event detection, anomaly identification, and forecasting. However, these techniques still face significant challenges in the online environment settings, encompassing aspects like efficiency, accuracy, robustness, and scarcity of labeled data. This dissertation presents four innovative solutions: FilCorr, DCT-MASS, FewSig, and BitLINK to overcome these challenges. We evaluate each method and showcase their practical significance through applications in earthquake early warning systems, aftershock sequence detection, and blockchain address identification. We hope this research will enhance our understanding of the challenges and opportunities in online temporal data mining and learning, ultimately leading to more efficient and robust algorithms.
Language
English
Keywords
Time series, Streaming, Distance Profile, Online Learning, Earthquake, Bitcoin
Document Type
Dissertation
Degree Name
Computer Science
Level of Degree
Doctoral
Department Name
Department of Computer Science
First Committee Member (Chair)
Abdullah Mueen
Second Committee Member
Shuang Luan
Third Committee Member
Bruna Jacobson
Fourth Committee Member
Jessica Lin
Recommended Citation
Zhong, Sheng. "Online Temporal Data Mining and Learning: Pursuing Enhanced Efficiency and Robust Algorithms." (2024). https://digitalrepository.unm.edu/cs_etds/126
Included in
Artificial Intelligence and Robotics Commons, Databases and Information Systems Commons, Data Science Commons, Geophysics and Seismology Commons