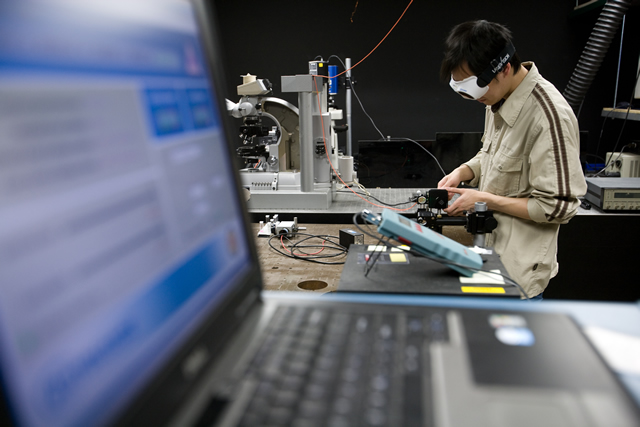
Computer Science ETDs
Publication Date
Spring 2-10-2023
Abstract
Positive and Unlabeled (PU) learning problems abound in many real-world applications. In healthcare informatics, diagnosed patients are considered labeled positive for a specific disease, but being undiagnosed does not mean they can be labeled negative. PU learning can improve classification performance, and estimate the positive fraction, α, among unlabeled samples. However, algorithms based on the Selected Completely At Random (SCAR) assumption are inadequate when the SCAR assumption fails (e.g., severe cases overrepresented), and when class imbalance is substantial. This dissertation presents and evaluates new algorithms to overcome these limitations. The proposed methods outperform the state-of-art for α-estimation, enhance classification performance, and provide well-calibrated classification on synthetic and benchmark datasets to support good decision thresholds. Furthermore, as verified through chart review, the proposed methods can detect uncoded self-harm events in electronic health records, and accurately estimate their prevalence, with demonstrated pharmacovigilance applications in mental health informatics.
Language
English
Keywords
positive and unlabeled learning, PU learning, noisy labels learning, machine learning, healthcare informatics, SCAR, SNAR, PULSNAR
Document Type
Dissertation
Degree Name
Computer Science
Level of Degree
Doctoral
Department Name
Department of Computer Science
First Committee Member (Chair)
Christophe G. Lambert
Second Committee Member
Abdullah Mueen
Third Committee Member
Trilce Estrada
Fourth Committee Member
Tudor I. Oprea
Project Sponsors
Patient‐Centered Outcomes Research Institute, NIH National Institute of Mental Health
Recommended Citation
Kumar, Praveen. "Machine Learning Methods for Computational Phenotyping Using Patient Healthcare Data with Noisy Labels." (2023). https://digitalrepository.unm.edu/cs_etds/116
Included in
Artificial Intelligence and Robotics Commons, Biomedical Informatics Commons, Medical Pharmacology Commons, Psychiatry and Psychology Commons, Theory and Algorithms Commons