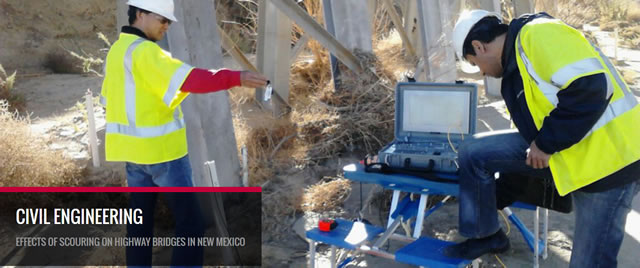
Civil Engineering Faculty Publications
Document Type
Article
Publication Date
2021
Abstract
The role of occupants in building energy management is well established. Occupant information from commercial buildings can function as a metric for the heating, ventilation, and air conditioning (HVAC) loads. Predicting these loads ahead in time could aid in setting up HVAC protocols to minimize wasteful energy demand and improve occupant comfort. To this extent, several frameworks were proposed that use occupancy data collected from commercial office buildings and graduate student offices to predict occupancy. However, the occupancy data collected in these frameworks are controlled and have limited complexity compared to larger commercial spaces such as airports, libraries, lecture halls, and shopping malls. Therefore, this paper presents the results of a study that demonstrates the feasibility of occupancy predictions from a relative dynamic environment using WiFi data and neural networks. The results demonstrate that reliable occupancy predictions are possible using intrinsic variables that have significant correlation with the occupancy profile of a time-segment. Similar conclusions can be extended to extrinsic variables if they are significantly correlated to the occupancy.
Recommended Citation
Jagadeesh Simma, Krishna Chaitanya; Thomas P. Caudell; and Susan M. Bogus. "Neural Network Based Occupancy Prediction using Patterns detected in WiFi Occupancy." (2021). https://digitalrepository.unm.edu/ce_fsp/2