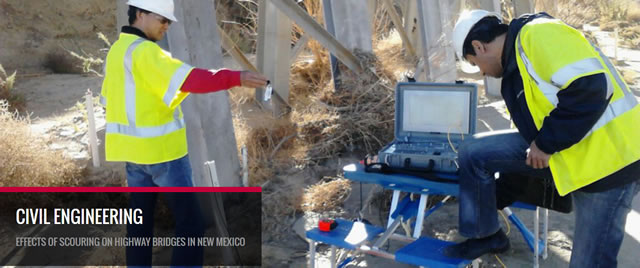
Civil Engineering ETDs
Publication Date
Fall 7-30-2024
Abstract
Improving road traffic safety relies on the ability to accurately predict traffic crashes and understand the factors contributing to severe injuries. This study employs a dual approach, utilizing both statistical analysis and machine-learning methodologies. Initially, statistical analyses such as chi-squared tests and Cramer's V values are applied to a decade's worth of police report data from Albuquerque, New Mexico, to identify primary causal factors behind crashes. Subsequently, machine-learning models are developed and rigorously compared to predict the severity of traffic crash injuries. The selection of the most effective algorithm is based on comprehensive metrics including accuracy, precision, recall, and F1 score, along with a detailed examination of corresponding confusion matrices. It's crucial to recognize that this predictive model is not static; ongoing updates and retraining using fresh traffic crash data are imperative to capture evolving dynamics in road infrastructure, driver behavior, and pedestrian interactions. While the current model is tailored to Albuquerque, its broader utility necessitates adaptation and validation using pertinent traffic accident data from other geographic regions.
Keywords
Road Traffic Safety, Predictive Modeling, Statistical Analysis, Machine Learning, Traffic Crash Severity
Document Type
Thesis
Language
English
Degree Name
Civil Engineering
Level of Degree
Masters
Department Name
Civil Engineering
First Committee Member (Chair)
Susan Bogus Halter
Second Committee Member
Su Zhang
Third Committee Member
Lisa Losada Rojas
Recommended Citation
Golrokh Amin, Arian. "EVALUATION OF MACHINE LEARNING ALGORITHMS AND STATISTICAL ANALYSIS FOR PREDICTING CRASH SEVERITY AND DETERMINING CONTRIBUTING FACTORS: A COMPARATIVE STUDY." (2024). https://digitalrepository.unm.edu/ce_etds/340