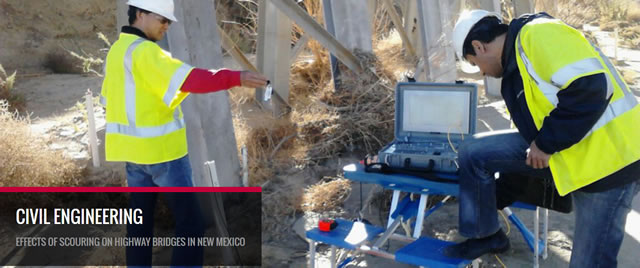
Civil Engineering ETDs
Publication Date
Summer 8-1-2023
Abstract
In the past decade, high-frequency water quality sondes have become more abundant in watersheds across North America and Europe and are gaining a foothold in Asia and South America. In this dissertation, three relevant topics associated with high-frequency data are investigated, i.e., the impact of winter’s precipitation on surface water quality and stream metabolism, the longitudinal propagation of wildfire disturbances through a fluvial network, and the use of machine learning with high-frequency data to estimate fluvial nutrient processing. First, we found that significant snow precipitation can cause surface water anoxia and declines in stream metabolism. Second, our data illustrate that fluvial water quality and metabolic activity degradation can propagate hundreds of kilometers downstream from a wildfire. Lastly, our work demonstrates that recurrent neural networks can outperform traditional regression methods when using atmospheric parameters to estimate nitrate uptake.
Keywords
Fluvial, High-Frequency, Water Quality, Stream Metabolism
Document Type
Dissertation
Language
English
Degree Name
Civil Engineering
Level of Degree
Doctoral
Department Name
Civil Engineering
First Committee Member (Chair)
Ricardo González-Pinzón
Second Committee Member
David Van Horn
Third Committee Member
José M. Cerrato
Fourth Committee Member
Laura J. Crossey
Recommended Citation
Nichols, Justin Rae. "Combining Semi-Continuous and Discrete Water Quality Data to Characterize Solute Transport, Biogeochemical Processes, and Wildfire Disturbances in Fluvial Systems." (2023). https://digitalrepository.unm.edu/ce_etds/310
Included in
Civil and Environmental Engineering Commons, Environmental Monitoring Commons, Hydrology Commons