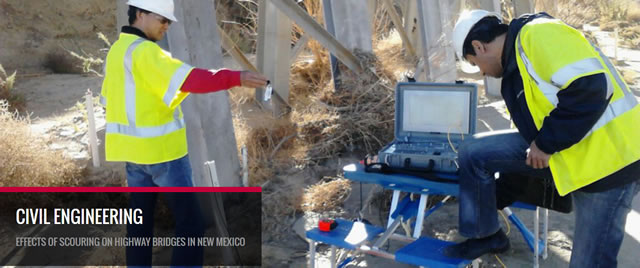
Civil Engineering ETDs
Publication Date
Summer 7-10-2021
Abstract
With the rise of technology use in buildings, it is now possible to collect data that can be used to improve building energy consumption. One factor that has significant impact on building energy consumption is occupancy. Recent studies have shown promising results in obtaining occupancy information from existing infrastructure such as WiFi router networks. However, these existing frameworks require additional investments through software upgrades, added infrastructure, computational resources, and may raise occupant privacy concerns. Additionally, with occupant thermal comfort statistics being lower than ASHAREA specified standards, a novel approach for indoor climate control is needed. To address the limitations in existing frameworks and the lower occupant thermal comfort statistics, this study proposes a framework to estimate occupancy from existing WiFi network data with minimal computational efforts and reduced privacy concerns. The structure of the occupancy data was studied to learn patterns within the data. The learned patterns are then used to make short term occupancy profile predictions using neural networks. The WiFi measured occupancy data showed a correlation up to 0.96 implying that WiFi client-count can be a reliable source of occupant count. HVAC energy consumption values were estimated using simulation models developed in EnergyPlus for the lecture hall used for data collection. The HVAC energy consumption for different occupancy-based schedules was estimated and compared against a fixed schedule (typical 6am-6pm operation assuming full occupancy), and a registered schedule (using total number registered occupants per each lecture scheduled in the lecture hall). The energy consumption results suggest that occupant demand-driven HVAC operation can results in energy savings of 50% compared to the fixed schedules.
The results from the occupancy pattern study revealed that by dividing the 24-hour occupancy profiles into smaller segments bound by external schedules, significant patterns can be learned. In this study, the external schedules were defined by the length of lectures scheduled for the Fall 2019 semester. Additionally, each time-segment will have at least one frequently occurring pattern labeled as Most Likely Template (MLT) and a pattern with zero-occupancy profiles labeled as Holiday Template (HT). The MLT patterns identified per time-segment can serve as expected occupancy for that time-segment. However, the different patterns learned for each time-segment suggest that using MLT profiles for expected occupancy may not always be correct. Therefore, a prediction mechanism was devised to avoid incorrect profile assignments for a time-segment. Using the patterns learned and neural networks (Laterally Primed Adaptive Resonance Theory, LAPART), short term occupancy profile predictions were made. The results from the prediction analysis imply that reliable occupancy prediction is possible if there are intrinsic or extrinsic variables with significant correlation to occupancy. The forecast of occupant count can be used for pre-conditioning the space personalized to the number of occupants in the space (i.e., establishing thermal setpoints as a function of occupant count) which can increase occupants’ thermal comfort and reduce unnecessary HVAC demand.
Keywords
HVAC, Energy, Occupancy, Neural Networks, Prediction, Energy Efficiency
Document Type
Dissertation
Language
English
Degree Name
Civil Engineering
Level of Degree
Doctoral
Department Name
Civil Engineering
First Committee Member (Chair)
Susan Halter Bogus
Second Committee Member
Fernando Moreu
Third Committee Member
Thomas P Caudell
Fourth Committee Member
Amy Ballard
Recommended Citation
Simma, Krishna Chaitanya Jagadeesh. "Dynamic HVAC Energy Management Using Commercial Building Occupancy Metrics & Neural Networks." (2021). https://digitalrepository.unm.edu/ce_etds/290