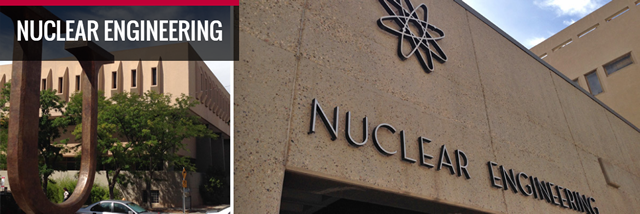
Nuclear Engineering ETDs
Publication Date
Spring 1-31-2019
Abstract
In the first study, a novel wireless pebble for scale experiments is developed, and a simple heat transfer experiment is conducted to determine the difference in the local heat transfer coefficient. Based on the fact that the use of Dowtherm A between approximately 57–87 °C is an alternative to the normal use of the FliBe temperature range of 600–700°C, a new-concept wireless device in a scaled experiment is introduced. This device consists of a 63.5 mm diameter metal shell and contains a built-in customized circuit board and battery for driving temperature measurements and wireless data transfer. The circuit board used for receiving temperature measurements from several type K thermocouples is based on the ATmega328 microprocessor (MCU). This board collects temperature at multiple points and send data to the receiver wirelessly. Also, the results from the experiment on the wireless communication between multiple devices are presented. The single wireless pebble did not change the value of the averaged heat transfer coefficient, even when the airflow rate changed and the attachment structure of Thermocouple (TC) was changed. Because the averaged heat transfer coefficient did not change significantly in the orientation of the internal heater, it could easily expand into several wireless pebbles. Lastly, the demonstration of three multiple wireless pebbles indicates that our suggested wireless pebbles can greatly help to estimate the local heat transfer coefficient. The results of the validation process will be extended to multiple wireless pebbles in future packed pebble-beds. This research is applicable to a case study for scaled experiments with packed pebble-beds for fluoride salt-cooled high-temperature reactors. We expect that this study will provide an experimental basis for pebbles for those who wish to use a non-invasive wireless device, which offers a powerful approach to investigating heat transfer coefficients in a non-invasive manner and to designing randomly packed configurations for further studies.
In the second study, a prediction of accident diagnosis using machine learning was performed. The simple case of an unprotected loss of flow accident (LOFA) was selected for simulation for a fuel pin at the start of different flow rates. The obtained outlet temperatures were used to identify the relationship between peak fuel temperatures and flow rate changes using a support vector machine (SVM). The SVM, trained with the core outlet temperature, provided an accurate prediction (R2 > 0.9) for changes in mass flow rate and peak surface temperature cladding in the early phase of LOFA transience (~0.5 s) unless the amount of training data was limited.
This illustrates that the key accident features are reflected well in the reactor core’s early response (i.e., core outlet temperature). This implies two possibilities. The first is to implement a different diagnostic framework from the current behavioral response to a prolonged progression of the accident. The second is to provide effective guidance for accident mitigation strategies in the early stages of accident progression.
The high predictability (i.e., R2 > 0.9) presented in the early phase of unprotected LOFA shows that the core outlet temperature is strongly correlated with both the change in flow rate and the peak surface cladding temperature throughout the entire transience. This strong correlation between different physical parameters allows for the possibility of interdependent detector systems by reducing the traditional boundaries of physical location and physical quantities in accident response and progress detection.
Keywords
Wireless pebble, packed-Bed FHR, Support Vector Machine, Machine Learning
Sponsors
University of New Mexico’s Nuclear Engineering Department
Document Type
Thesis
Language
English
Degree Name
Nuclear Engineering
Level of Degree
Masters
Department Name
Nuclear Engineering
First Committee Member (Chair)
Prof. Youho Lee
Second Committee Member
Prof. Cassiano R. E. de Oliveira
Third Committee Member
Prof. Sang Lee
Recommended Citation
Chang, Dongjune. "DEVELOPMENT OF WIRELESS PEBBLE FOR PACKED BED HEAT TRANSFER MEASUREMENTS AND MACHINE LEARNING-AIDED ACCIDENT DIAGNOSIS FOR LOSS OF FLOW ACCIDENT (LOFA)." (2019). https://digitalrepository.unm.edu/ne_etds/81