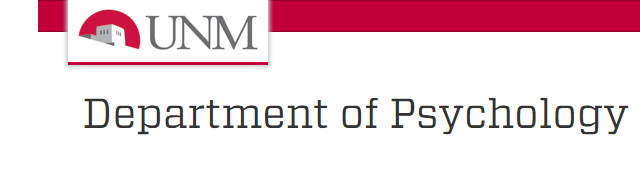
Psychology ETDs
Publication Date
8-25-2016
Abstract
In a longitudinal study, the data can be either time-structured, where the times of assessment are the same across participants, or time-unstructured, where the times of assessment vary across participants. Currently, growth curve modeling can accommodate time-unstructured data when modeling average change over time (fixed effects) and variability in change over time (random effects), but not residual variances and covariances. Through Monte Carlo simulation, a first goal of this study was to determine the effects of ignoring variability in times of assessment when modeling the residual variances and covariances in linear growth curve models in terms of convergence, parameter bias, power to detect change over time, and model fit. A second goal was to evaluate a novel method to construct partially' time-unstructured matrices for residual variances and covariances. The simulation factors manipulated in this study are type of analysis (time-structured, partially time-unstructured, fully time-unstructured), residual matrix (heterogeneous diagonal, first-order autoregressive), sample size (50, 200, 500), and number of time points (3, 6, 9). Results showed that convergence was generally high when the matrix was autoregressive, whereas when the matrix was heterogeneous diagonal, the time-structured and partially time-unstructured analyses converged most often; in many conditions, the fully time-unstructured analysis never converged. Fixed effects were generally spared from bias across conditions, as were the random effects when the matrix was heterogeneous diagonal. With the time-structured autoregressive matrix and only 3 time points, the intercept and slope variances were overestimated, and the intercept-slope correlation and residual variance were underestimated. There was a large effect of type of analysis on autocorrelation bias, with only the fully time-unstructured analysis yielding unbiased estimates, and the partially time-unstructured analysis yielding less bias than the time-structured analysis. Power to detect change over time was high across conditions. In terms of model fit, all fit indexes examined favored time-unstructured analyses when the matrix was autoregressive, whereas only deviance favored time-unstructured analyses when the matrix was heterogeneous diagonal. Overall, this study shows that accommodating time-unstructured data when modeling residual variances and covariances can be important, perhaps especially when residuals are autocorrelated. Moreover, when the fully time-unstructured matrix cannot be used, the partially time-unstructured matrix provides an improvement over the standard time-structured matrix under certain conditions.
Degree Name
Psychology
Level of Degree
Doctoral
Department Name
Psychology
First Committee Member (Chair)
Selig, James
Second Committee Member
Vigil, Jacob
Third Committee Member
Witkiewitz, Katie
Language
English
Keywords
growth curve, time-unstructured analysis, residual variance, level-1 matrix, misspecification, simulation, longitudinal
Document Type
Dissertation
Recommended Citation
Coulombe, Patrick. "Partially and fully time-unstructured residual variance-covariance matrices in growth curve modeling: Consequences of ignoring variability in times of assessment." (2016). https://digitalrepository.unm.edu/psy_etds/28