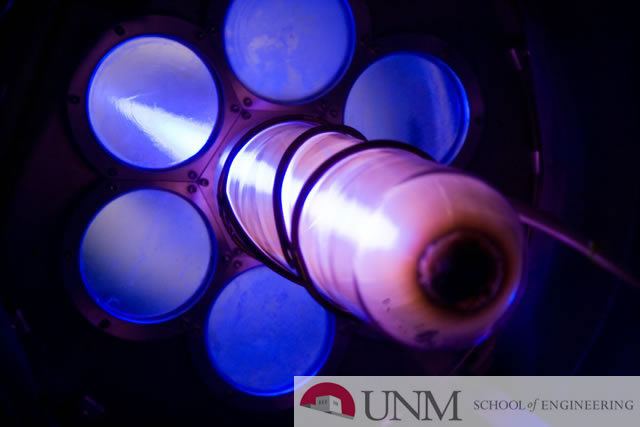
Electrical and Computer Engineering ETDs
Publication Date
Summer 8-1-2023
Abstract
Speaker diarization from a single microphone is extremely challenging in noisy classroom environments. A new method based on simulating a microphone array has shown promising results, while requiring very little training. It used a minimum distance classifier to identify the speaker among a list of possible speakers. This thesis investigates machine learning methods for determining the speaker. The AOLME dataset that was used contains 758 samples totaling 894.4 seconds. Each is taken from a noisy classroom environment, focusing on five speakers - any one of whom could be active in a given sample - lasting an average of 1.2 seconds. Data augmentation effectively doubled the samples in the data set. The machine learning schemes tested were a neural network, support vector machine, k nearest neighbors, random forests, gradient boosting and voting classifier integrating several of these. Our best performance of 86.4% classification accuracy was achieved with random forests.
Document Type
Thesis
Language
English
Degree Name
Computer Engineering
Level of Degree
Masters
Department Name
Electrical and Computer Engineering
First Committee Member (Chair)
Marios Pattichis
Second Committee Member
Xiang Sun
Third Committee Member
Ali Bidram
Fourth Committee Member
Tyler Lovelly
Recommended Citation
Briggs, Richard. "Speaker Diarization of Noisy Classrooms from a Single Microphone Based on an Array of Virtual Microphones and Machine Learning." (2023). https://digitalrepository.unm.edu/ece_etds/605